With the ever-growing surge in mobile apps, capturing attention from prospective users has never been more challenging.
Advertisers are now realizing the immense value in using AIArtificial Intelligence, systems that simulate human intelligence processes for ad targeting, discovering how it can drive up engagement rates and boost effectiveness.
AI-driven targeting across Google Ads has opened new doors to deeper audience insights and personalized user experiences.
Whether it’s ultra-specific targeting or predicting user behavior, integrating AI into targeting strategies elevates app marketing to new heights.
But what does it take to effectively harness AI for app ads, and what innovations are paving the way for better results?
In this article, we’ll explore the latest advancements in AI targeting and how you can leverage them to increase your app’s visibility and conversion rates.
- Understanding AI-Driven Targeting in Google Ads
- Targeting Personalization: The Road to Hyper-Relevant App Ads
- Predictive Analytics for Enhanced App User Engagement
- AI-Powered Contextual Targeting: How to Increase the Relevance of Ads?
- AI-Powered Ad Performance Metrics: How to Measure App Advertising Success
- Maximizing App Advertising with AI-Powered Targeting
- FAQs on AI-Powered Targeting for App Advertising
Understanding AI-Driven Targeting in Google Ads
AI-powered targeting in Google Ads isn’t just a passing trend; it’s revolutionizing how advertisers engage with their audience and optimize their budgets.
With AI, targeting is achieved with remarkable precision and efficiency.
AI not only speeds up data processing but also uncovers insights that traditional methods might miss.
By understanding how AI-driven targeting works, you can unlock a wealth of benefits that enhance user reach and improve your ad campaign’s ROIReturn on Investment, a measure of profitability relative to the investment cost.
AI’s potential to analyze vast amounts of data quickly and accurately is incredibly powerful.
Through machine learningA subset of AI that enables systems to learn from data and improve over time algorithms, Google Ads can learn from past campaigns and user behaviors, making it possible to reach the right audience at the perfect time.
AI takes into account hundreds of variables—from browsing habits to purchasing behavior—all in real-time.
The result?
Ads that are relevant, timely, and personalized for each user.
AI targeting goes beyond simple demographic parameters by incorporating behavior patterns, browsing preferences, and contextual factors.
This precision allows advertisers to create more sophisticated and fitting ad placements.
For example, if a user frequently searches for fitness apps, AI can display your health-related app ad at the optimal moment to capture their interest.
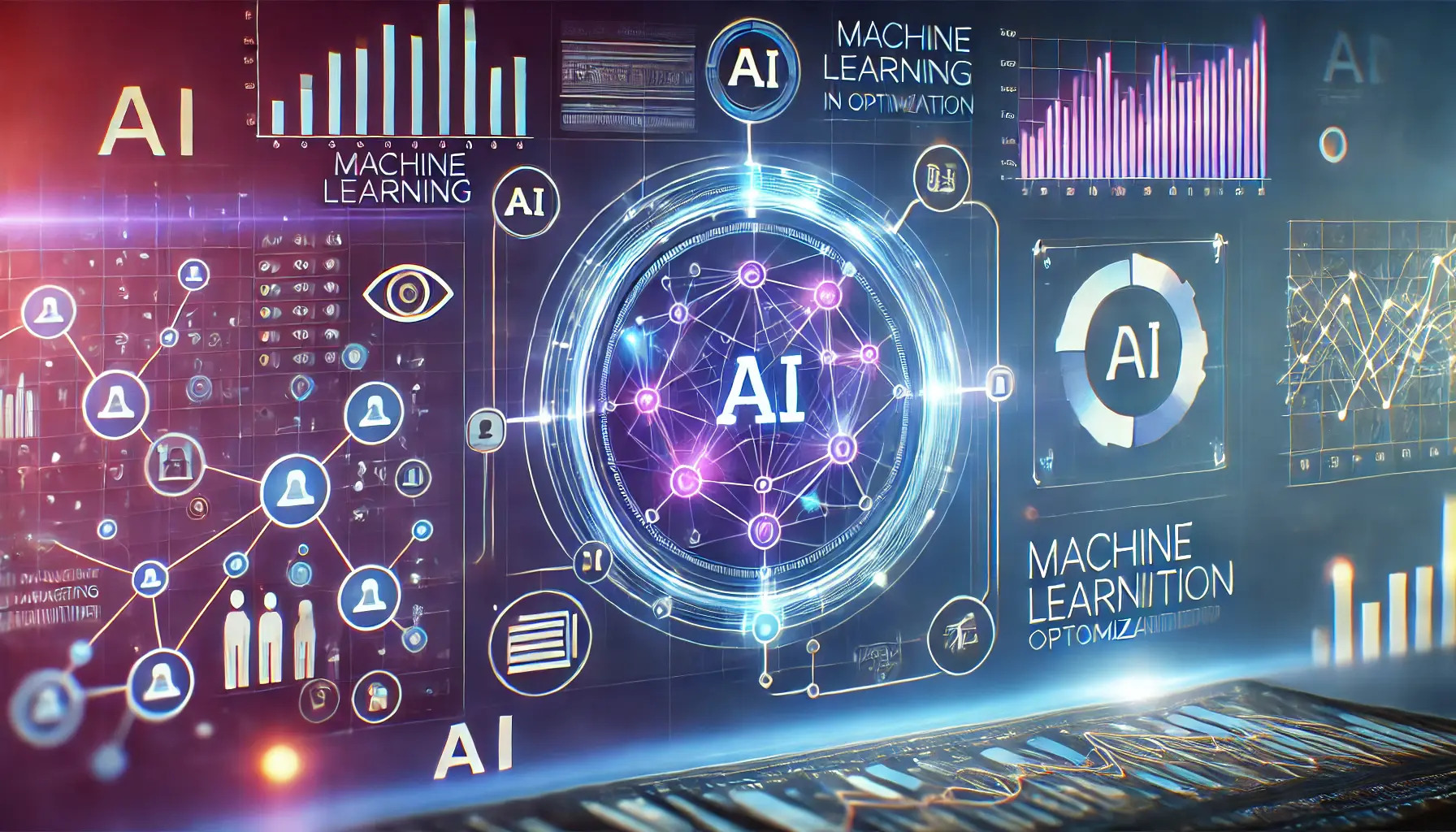
Machine learning enhances ad optimization through data-driven insights and real-time adjustments.
The Role of Machine Learning in Ad Optimization
Machine learning, a subset of AI, plays a critical role in ad optimization.
By analyzing historical ad performance data, machine learning algorithms continuously adapt to deliver better targeting over time.
Essentially, your ads become smarter with each impression.
Machine learning adjusts bids, placement, and even ad content based on real-time performance data, creating campaigns that are more responsive to user behavior.
Continuous optimization through AI-driven campaigns can yield impressive results without requiring constant manual adjustments.
This saves marketers time, allowing them to focus on strategic decisions that enhance overall campaign efficiency.
Furthermore, machine learning can help identify the ad placements most likely to convert, enabling advertisers to maximize their ad spend effectively.
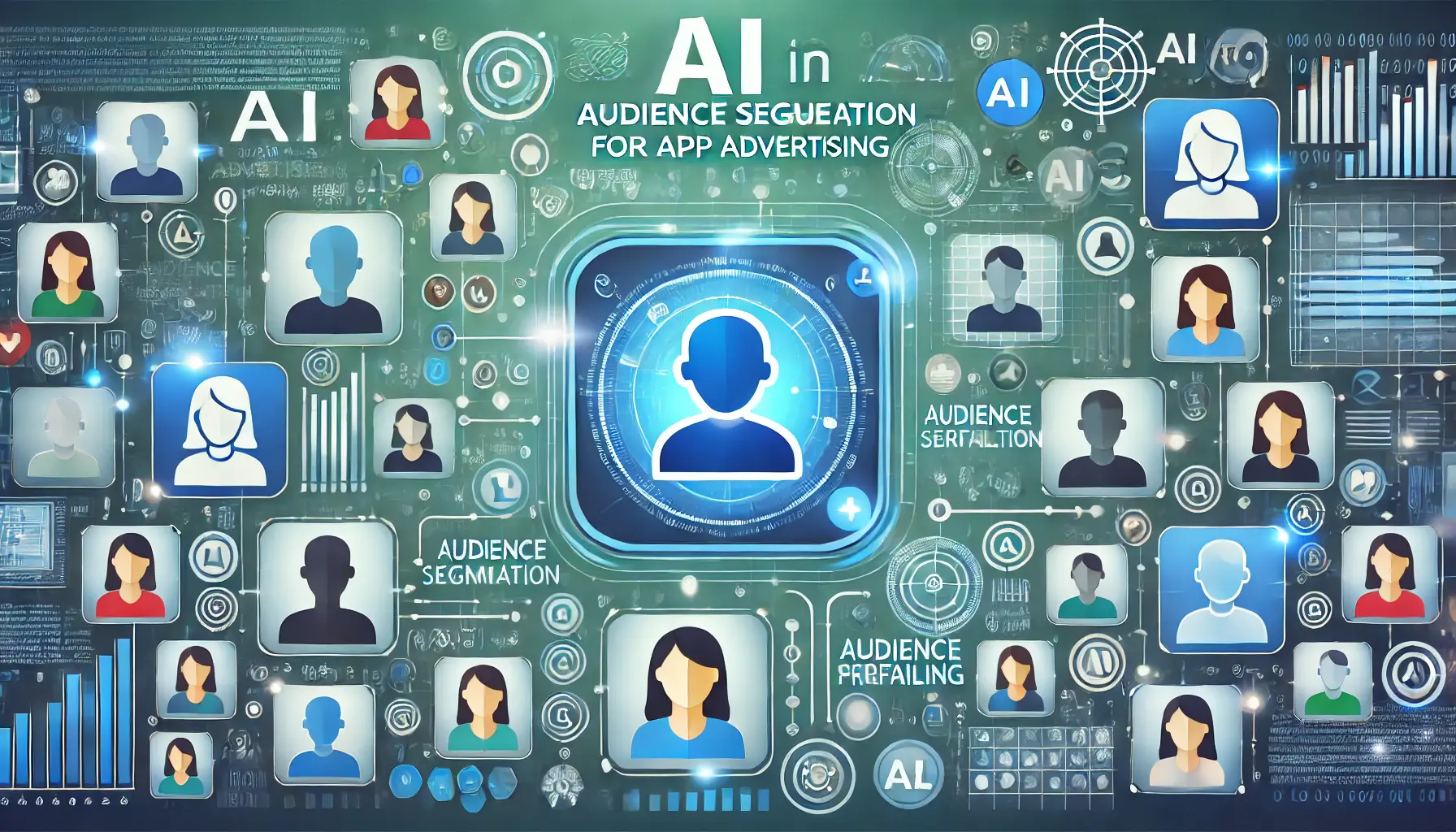
AI enhances audience segmentation, enabling precise targeting and personalization in advertising.
Benefits of AI in Audience Segmentation
Audience segmentationDividing a target audience into distinct groups based on shared characteristics is crucial for delivering relevant app ads, and AI is a game-changer in this area.
AI allows advertisers to segment their audience into highly targeted groups based on a range of factors such as behavior, interests, and demographics.
This level of segmentation enables advertisers to create personalized messages for each segment, improving user engagement and conversion rates.
For example, if your app serves different purposes for various user groups, AI can identify and target each group with tailored messages.
Whether it’s casual users or power users, AI-driven audience segmentation makes it easier to create ads that cater to each group’s unique needs and interests.
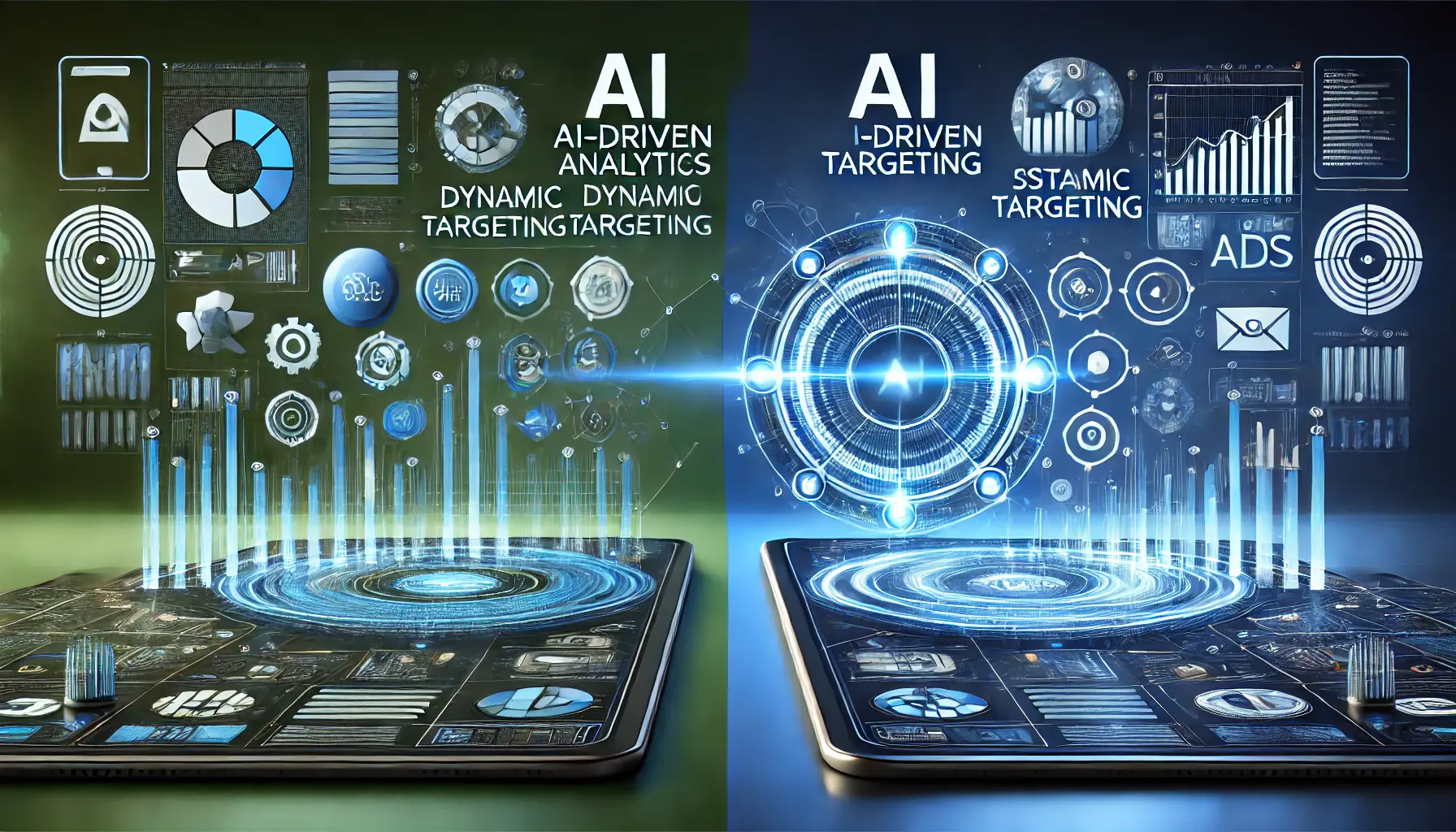
AI-driven ads offer advanced precision and engagement compared to traditional targeting methods.
Why AI-Driven Ads Outperform Traditional Targeting
AI-powered ads have a distinct advantage over traditional targeting methods due to their flexibility and data-driven approach.
Traditional methods often rely on assumptions or outdated historical data, while AI-driven ads use real-time data to ensure relevance and effectiveness even as trends and user preferences evolve.
AI-driven targeting allows advertisers to stay agile, adapting to shifts in user behavior almost instantly.
This results in more efficient strategies that maximize engagement and minimize ad spend waste.
By investing in AI-driven targeting, you not only extend your ad’s reach but also build a more sustainable and impactful advertising approach.
AI-driven targeting in Google Ads enhances precision by analyzing vast datasets, allowing ads to reach the right audience at the optimal time, maximizing engagement and efficiency.
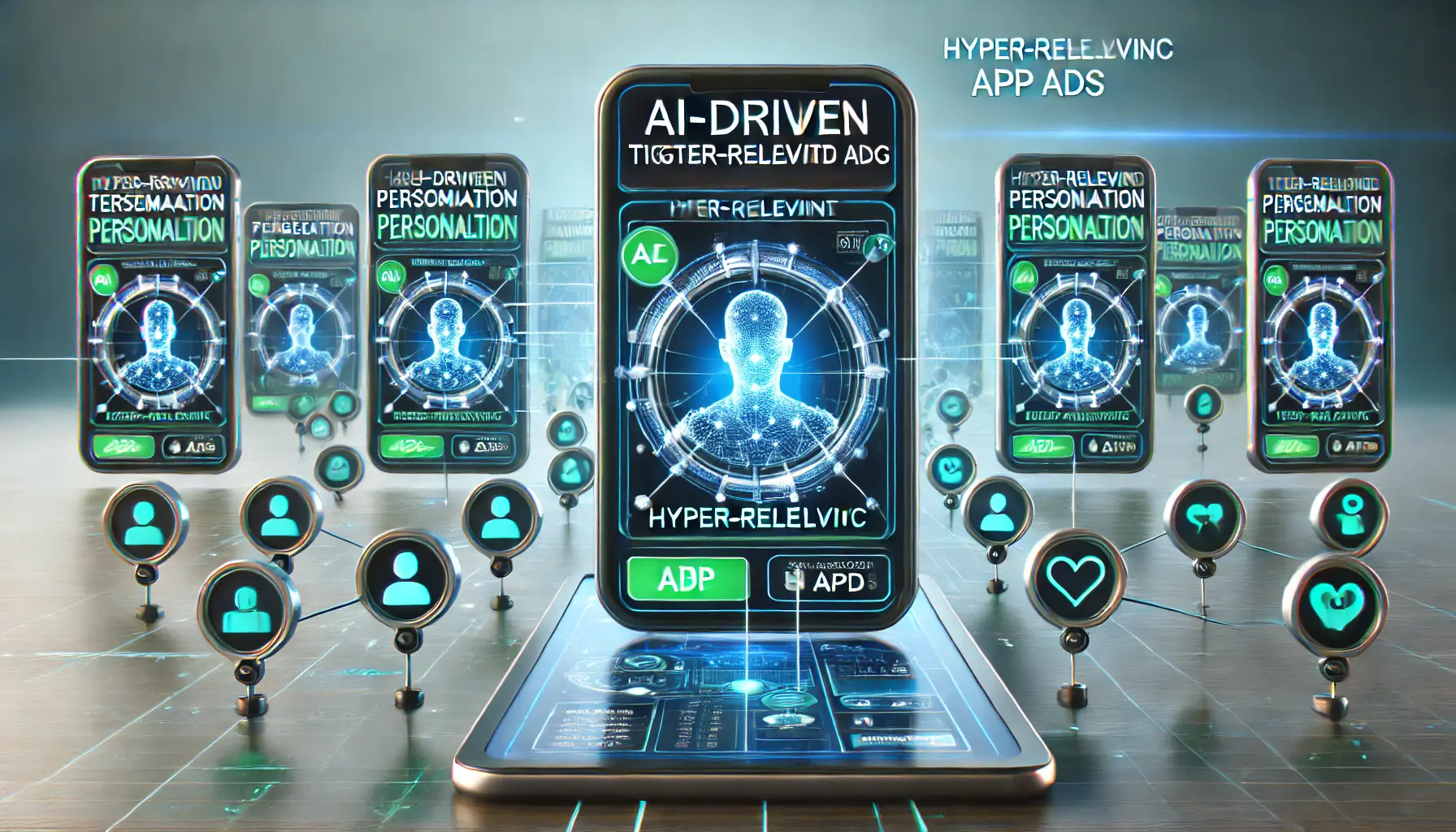
AI-driven targeting personalization enables hyper-relevant app ads tailored to individual user interests.
Targeting Personalization: The Road to Hyper-Relevant App Ads
Personalization is the buzzword in today’s digital advertising space.
Users are bombarded with numerous ads every day, and only the most relevant and curated ads stand out.
AI-driven personalized targeting enables advertisers to create hyper-relevant app ads, tailored specifically to each user’s tastes, behaviors, and interests.
With AI, advertisers can go beyond basic demographic targeting, diving deeply into individual user data to make each ad feel personal, timely, and relevant.
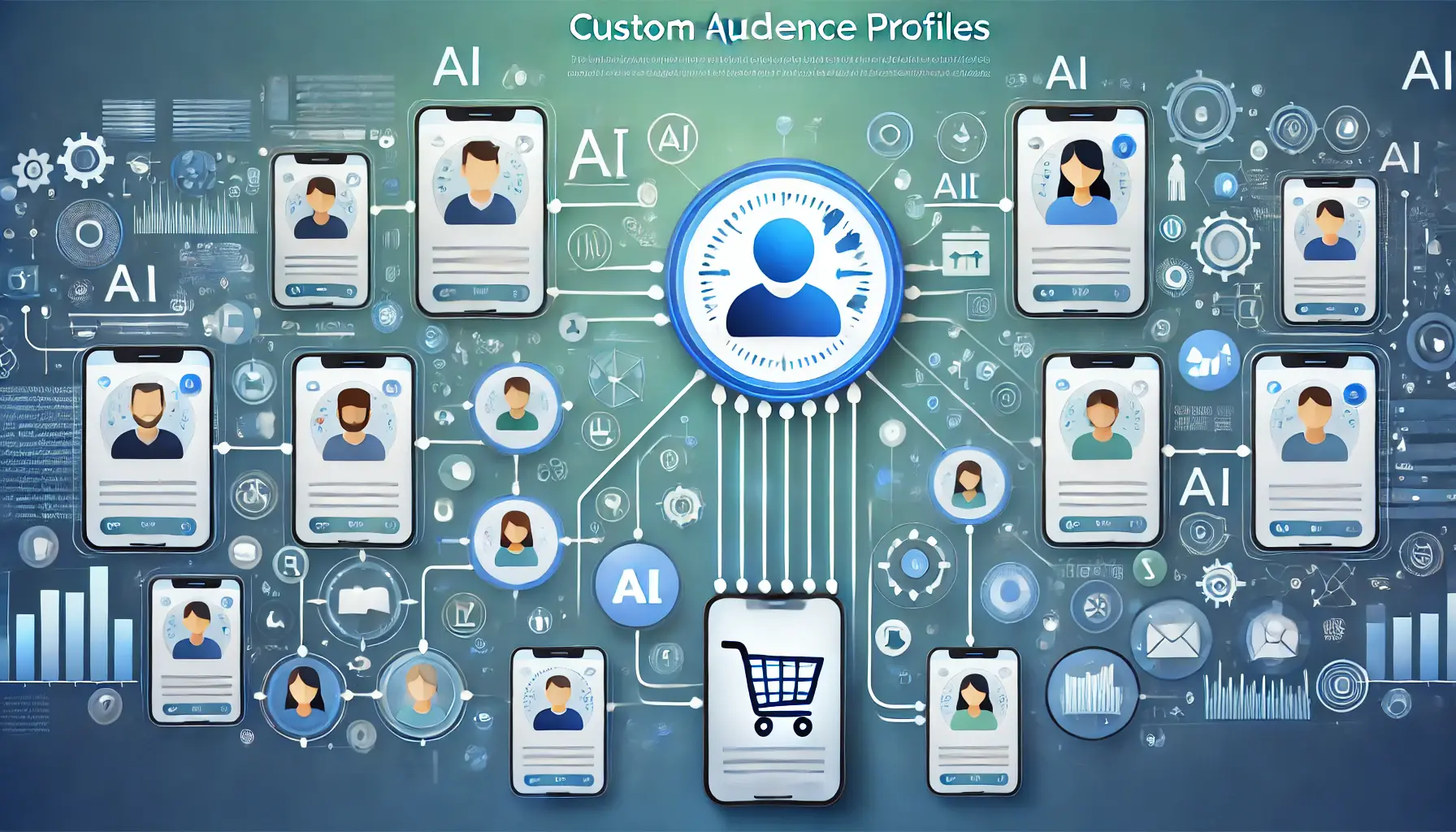
AI-driven audience profiling allows for the creation of highly customized user profiles for targeted app ads.
Creating Custom Audience Profiles with AI
One powerful way AI aids in personalized targeting is by building detailed audience profiles.
AI processes user data from multiple touchpoints, such as browsing history, app usage, and past purchases, to create comprehensive profiles.
These profiles allow advertisers to reach users with ads that are likely to interest them and generate engagement.
For example, if someone frequently engages with fitness-related content, AI will flag them as a potential target for health and wellness apps, creating highly relevant ad placement opportunities.
Custom audience profiles are particularly effective because they reflect a user’s preferences more accurately than general demographic data.
This specificity ensures that users see ads that are meaningful to them, which not only boosts engagement but enhances the overall user experience.
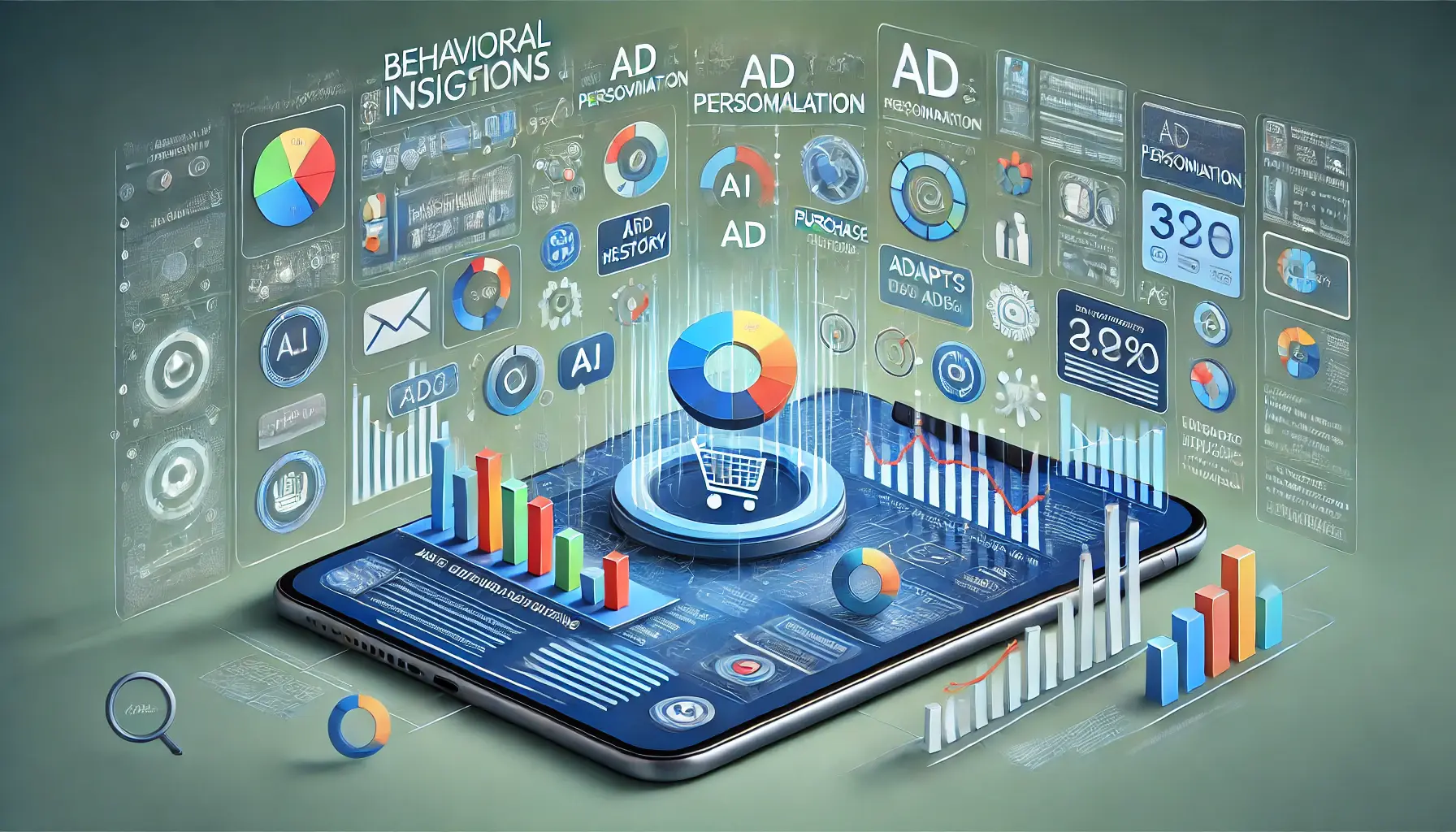
Behavioral insights are analyzed by AI to create personalized ad experiences based on user data.
Behavioral Insights for Ad Personalization
Behavioral insights are crucial to effective personalizationCustomizing content or ads based on individual user preferences or behaviors.
By analyzing user behavior—such as content interaction patterns, app visit frequency, and in-app screen time—AI provides insights that allow advertisers to design ads aligned with user behavior.
This approach enables advertisers to show app ads that relate to each user’s recent actions or interests.
- Engagement Patterns: AI tracks how users engage with different types of content, helping advertisers understand what truly appeals to them.
- Purchase Behavior: In e-commerce or subscription-based apps, AI analyzes purchase patterns, enabling advertisers to suggest similar or complementary products.
- Timing and Frequency: AI identifies when users are most active, helping ensure ads reach them at the optimal time.
These behavioral insights help target ads to the right users in a way that feels organic and engaging, driving higher conversions.
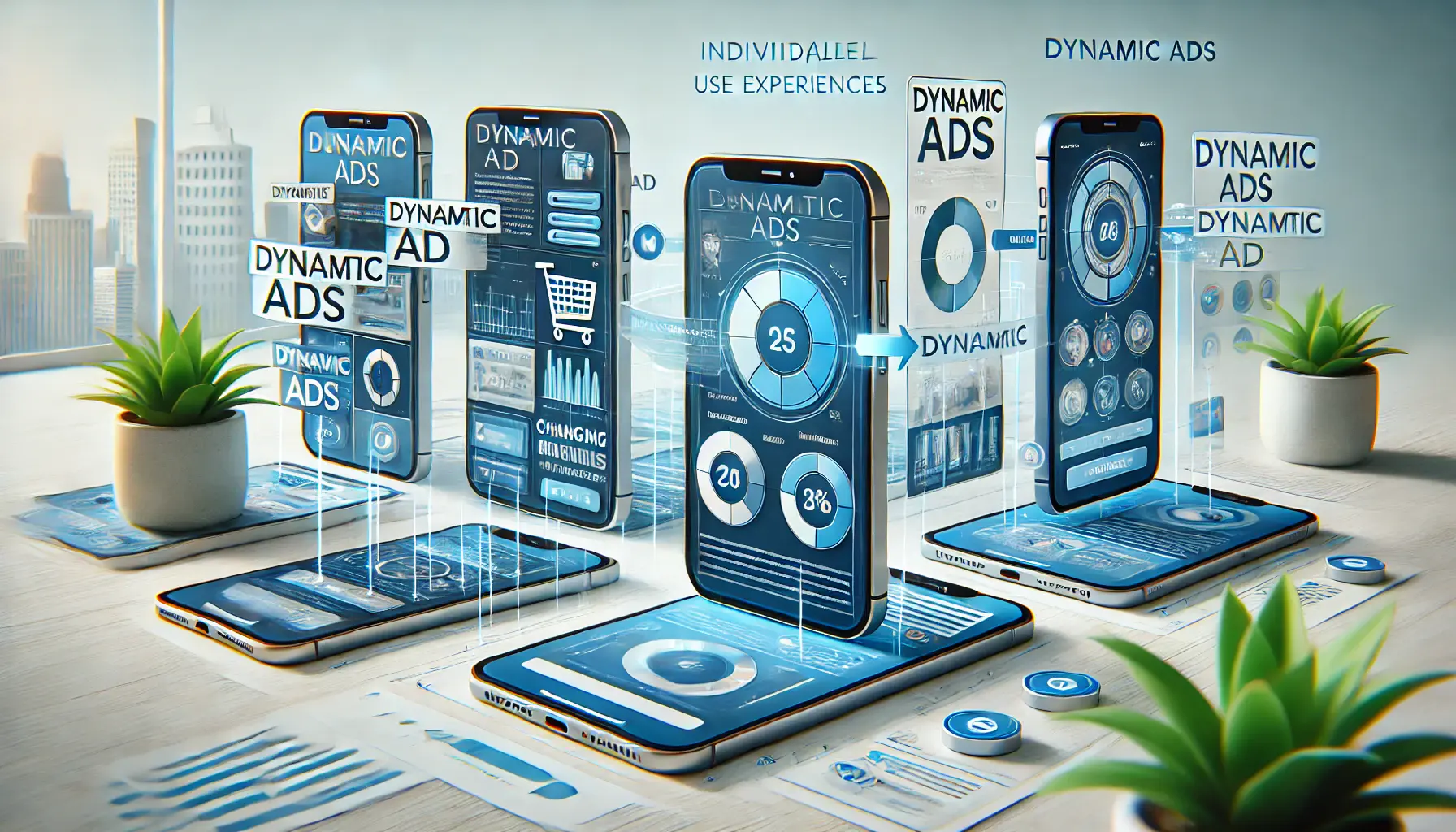
Dynamic ad formats adjust in real-time, providing personalized ad experiences tailored to individual user preferences.
Dynamic Ad Formats for Individualized Experiences
AI assists not only with audience selection but also with creating dynamic ad formats that adjust based on user data.
Dynamic ads provide a personalized experience by changing elements such as images, text, or call-to-action buttons based on the viewer.
For example, a dynamic ad for a travel app might display different destinations based on the user’s previous travel searches or preferences.
This flexibility in ad content makes each ad feel tailor-made for the viewer, increasing its relevance and boosting user interaction.
Dynamic ads are especially effective for mobile app ads, as they adjust to present content that resonates with each user, driving valuable interactions with your app.
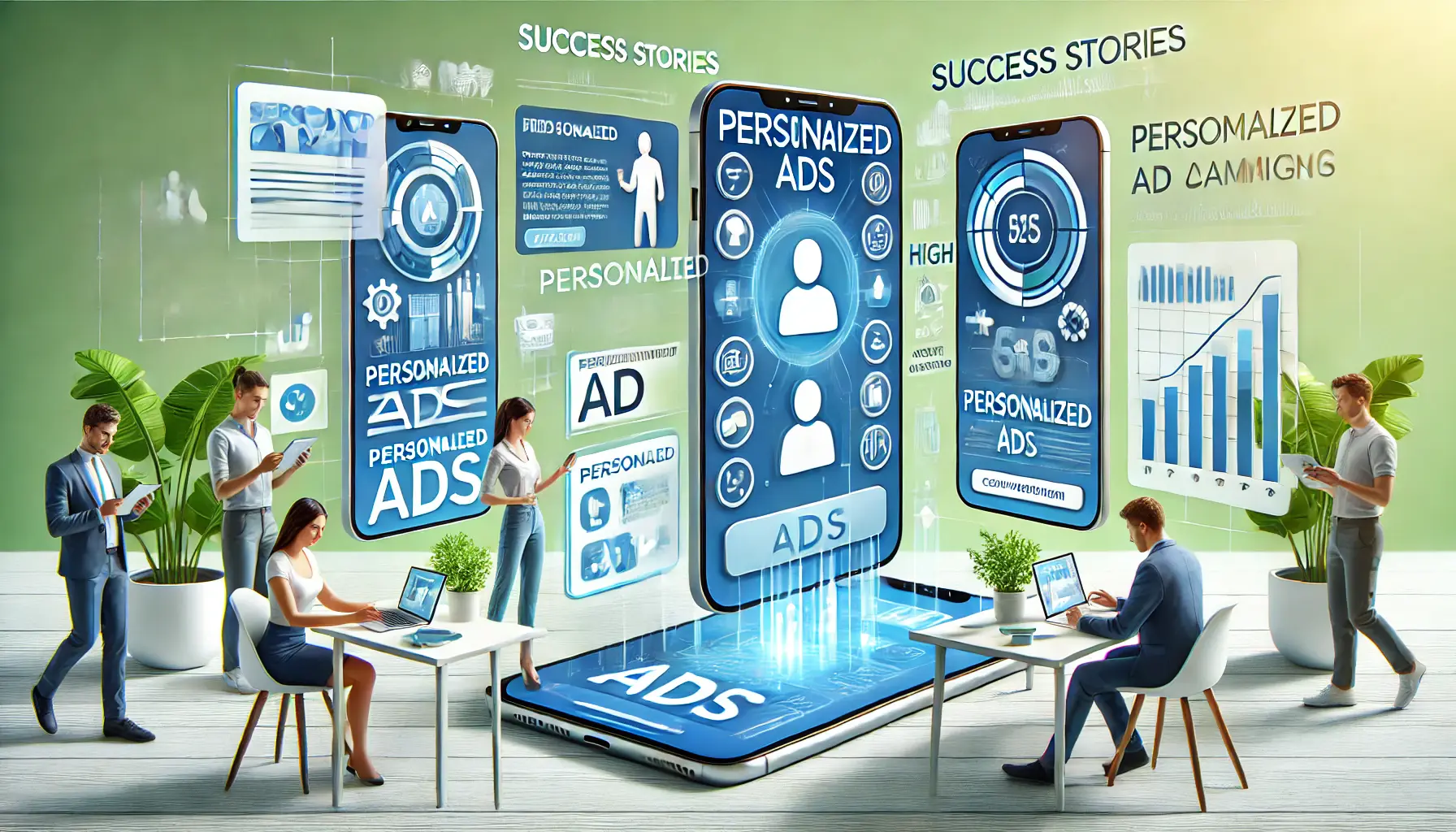
Personalized ads have led to significant success, increasing user engagement and conversions across various campaigns.
Success Stories of Personalized Ads
Several companies have leveraged AI to personalize app ads, achieving significant boosts in both engagement and ROI.
For instance, a leading streaming app used AI-based personalization to recommend new content based on users’ previous viewing history.
This approach resulted in a substantial increase in app engagement and time spent within the app.
Similarly, an e-commerce app increased conversions and reduced cart abandonment through AI-driven product recommendations.
These success stories highlight the power of personalized targeting in driving app success.
By applying AI to deliver hyper-relevant ads, brands can connect with users on a deeper level, leading to higher engagement, better conversion rates, and a more positive user experience overall.
Personalization through AI allows for hyper-relevant app ads that align with each user’s unique preferences, leading to higher engagement and more impactful ad experiences.
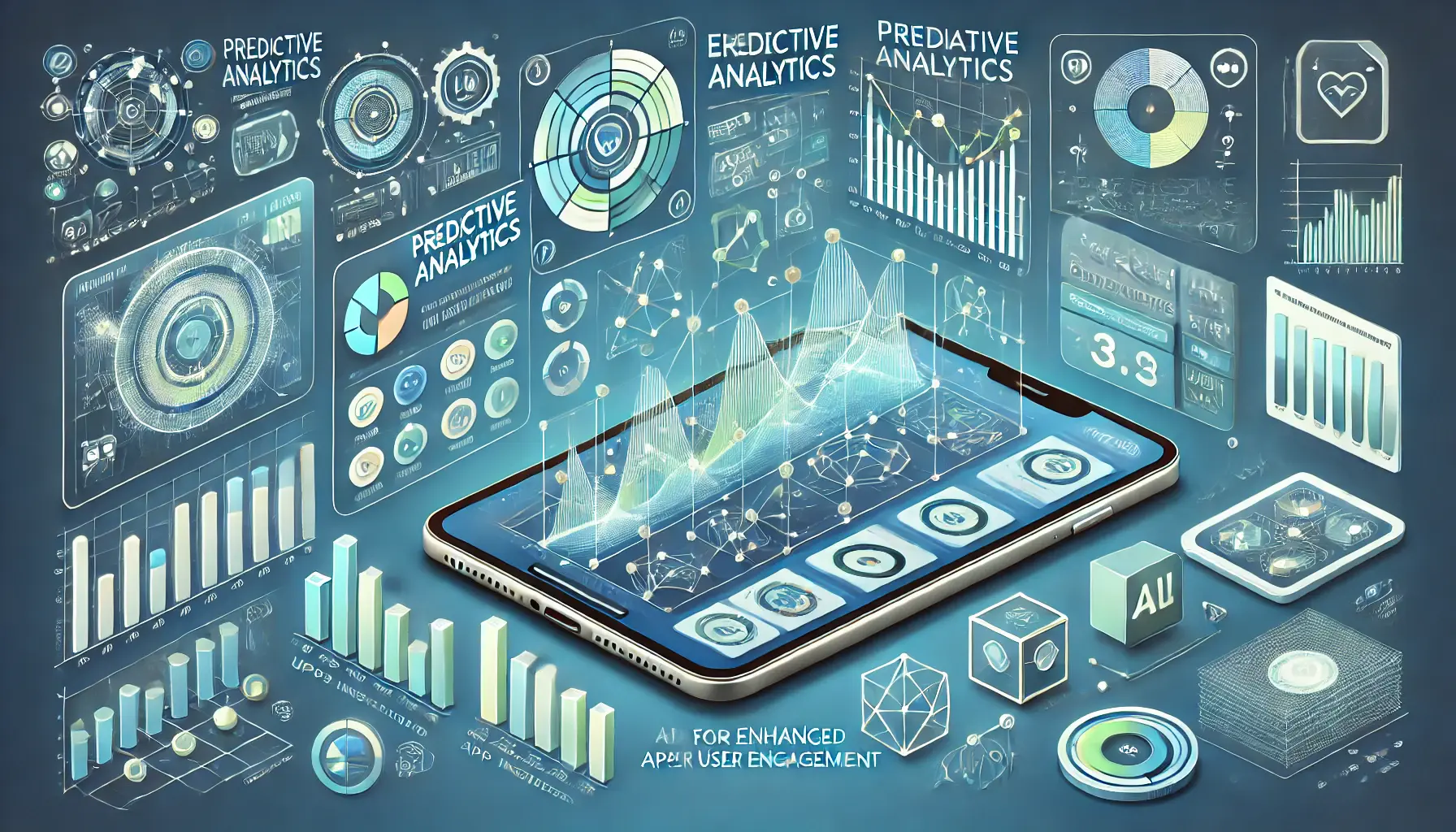
Predictive analytics enables accurate forecasting of user behavior, enhancing app engagement strategies.
Predictive Analytics for Enhanced App User Engagement
Predictive analytics represents the future of how advertisers engage with app users, enabling them to anticipate actions and preferences before they occur.
By analyzing vast amounts of data, predictive analyticsAnalyzing data to forecast future outcomes based on historical data forecasts what’s likely to happen next—such as app installs, purchases, or other content interactions.
These insights allow advertisers to create highly targeted campaigns that resonate with users, increasing engagement and retention rates.
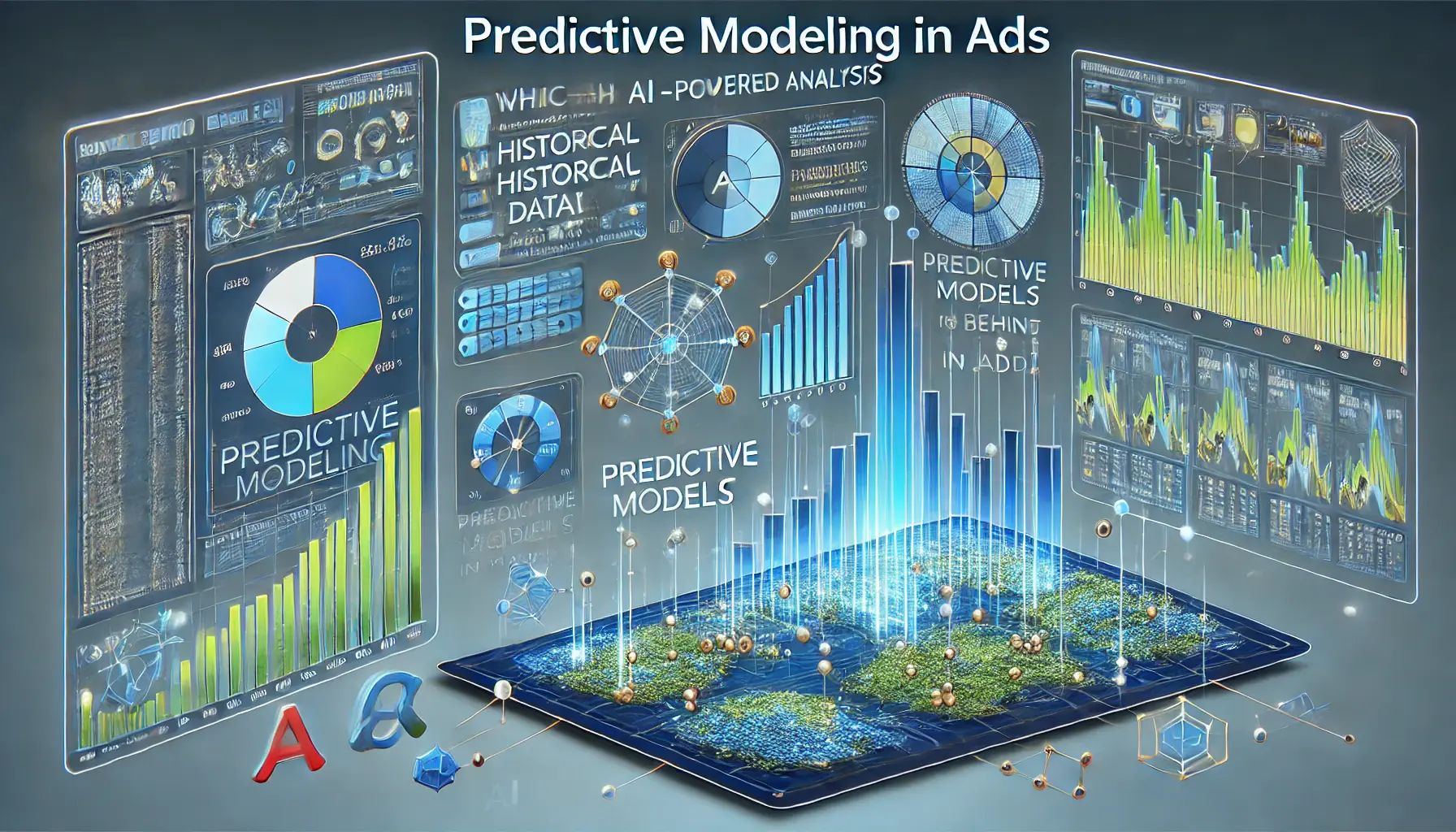
Predictive modeling in ads uses historical data to forecast user engagement and optimize targeting strategies.
Predictive Modeling in Ads
Predictive modeling relies on historical data to identify trends and patterns that drive forward-looking actions.
In app advertising, predictive models help pinpoint which users are most likely to engage with an ad or make an in-app purchase.
By analyzing past interactions, these models identify high-value user segments, delivering tailored ads that encourage further engagement.
For instance, if a user has shown interest in specific features of an app, predictive modeling can serve them ads that highlight similar features or benefits.
This personalization enhances re-engagement, making ad campaigns more effective overall.
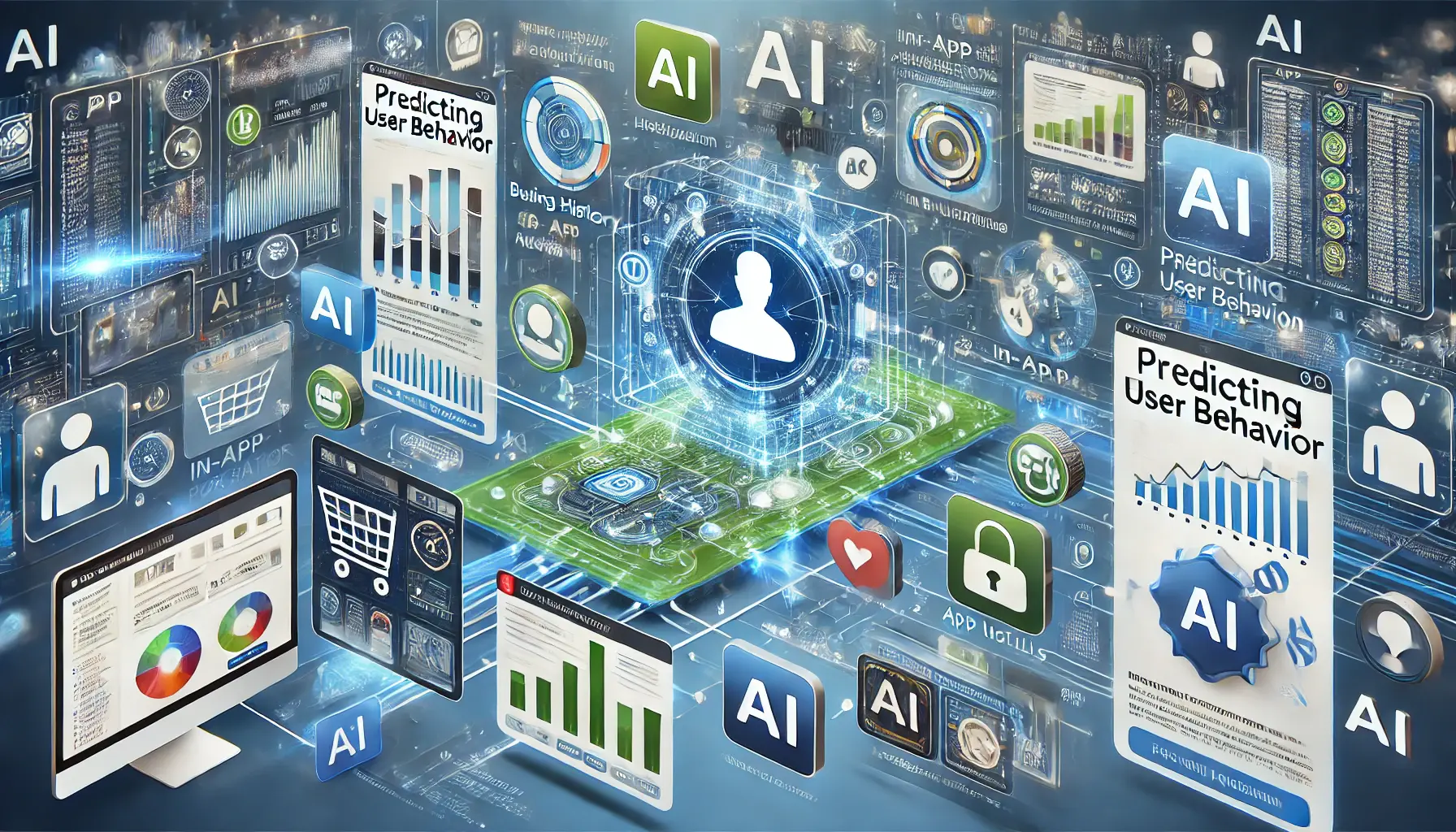
AI predicts user behavior by analyzing past interactions, improving app advertising strategies.
Predicting User Behavior with AI
AI-driven predictive analytics enables accurate predictions of user behavior, allowing advertisers to stay one step ahead.
This strategy analyzes browsing patterns, time spent on app screens, and previous purchases to determine the best times and formats for reaching users.
By understanding these patterns, predictive analytics makes it easier to predict when users may churn or when they’re likely to make an in-app purchase, allowing advertisers to adjust strategies accordingly.
- User Retention: Predictive analytics can identify users at risk of churning, allowing advertisers to serve re-engagement ads to retain them.
- Conversion Probability: By analyzing user actions, AI can estimate conversion probability, optimizing ad placements for maximum impact.
- Timing of Engagement: Forecasting tools determine optimal ad timing, ensuring ads reach users when they’re most likely to engage.
These insights enable predictive analytics to proactively drive user engagement through data-driven decisions, enhancing ad campaign performance and ROI.
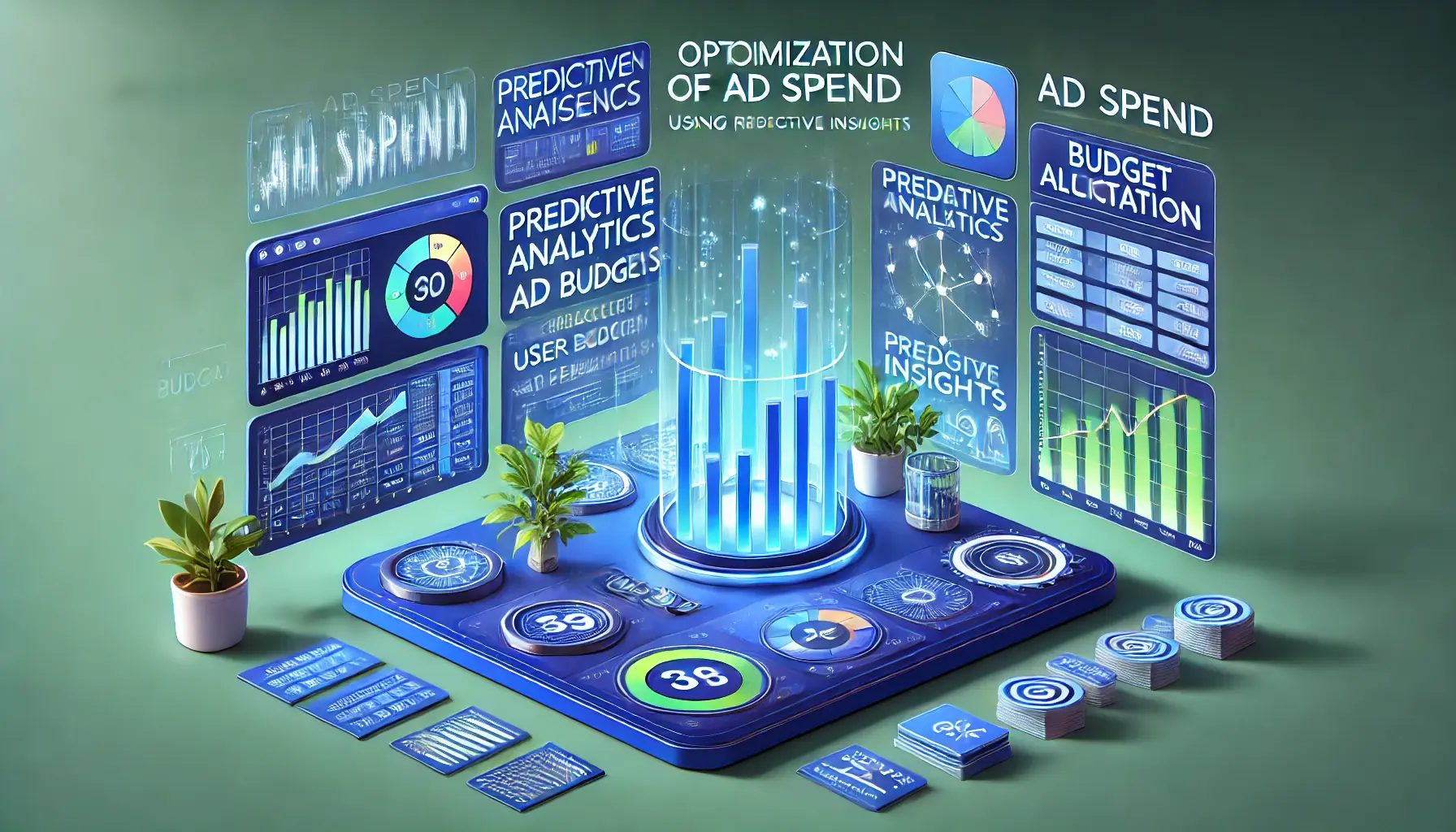
Predictive insights optimize ad spend by efficiently allocating budgets based on user behavior data.
Optimizing Ad Spend Using Predictive Insights
AI-powered predictive analytics is crucial for optimizing ad spend, allowing advertisers to allocate budgets efficiently by focusing on high-impact segments.
By identifying users who are likely to convert, predictive analytics minimizes ad spend waste and ensures that marketing dollars target users with the highest potential ROI.
Predictive analytics facilitates smarter budgeting, allowing advertisers to set precise goals and track performance metrics against predictive models.
This targeted approach enables advertisers to focus on campaigns that yield maximum value, leading to a more cost-effective advertising strategy.
For example, a food delivery app may use predictive modeling to identify users likely to order at specific times, leading to increased order frequency and customer retention.
Similarly, a mobile game developer uses predictive analytics to identify users likely to make in-game purchases, promoting relevant offers and exclusive content to drive engagement.
These examples demonstrate how predictive analytics improves advertising campaigns by allowing brands to anticipate user needs and actions, fostering better engagement and loyalty.
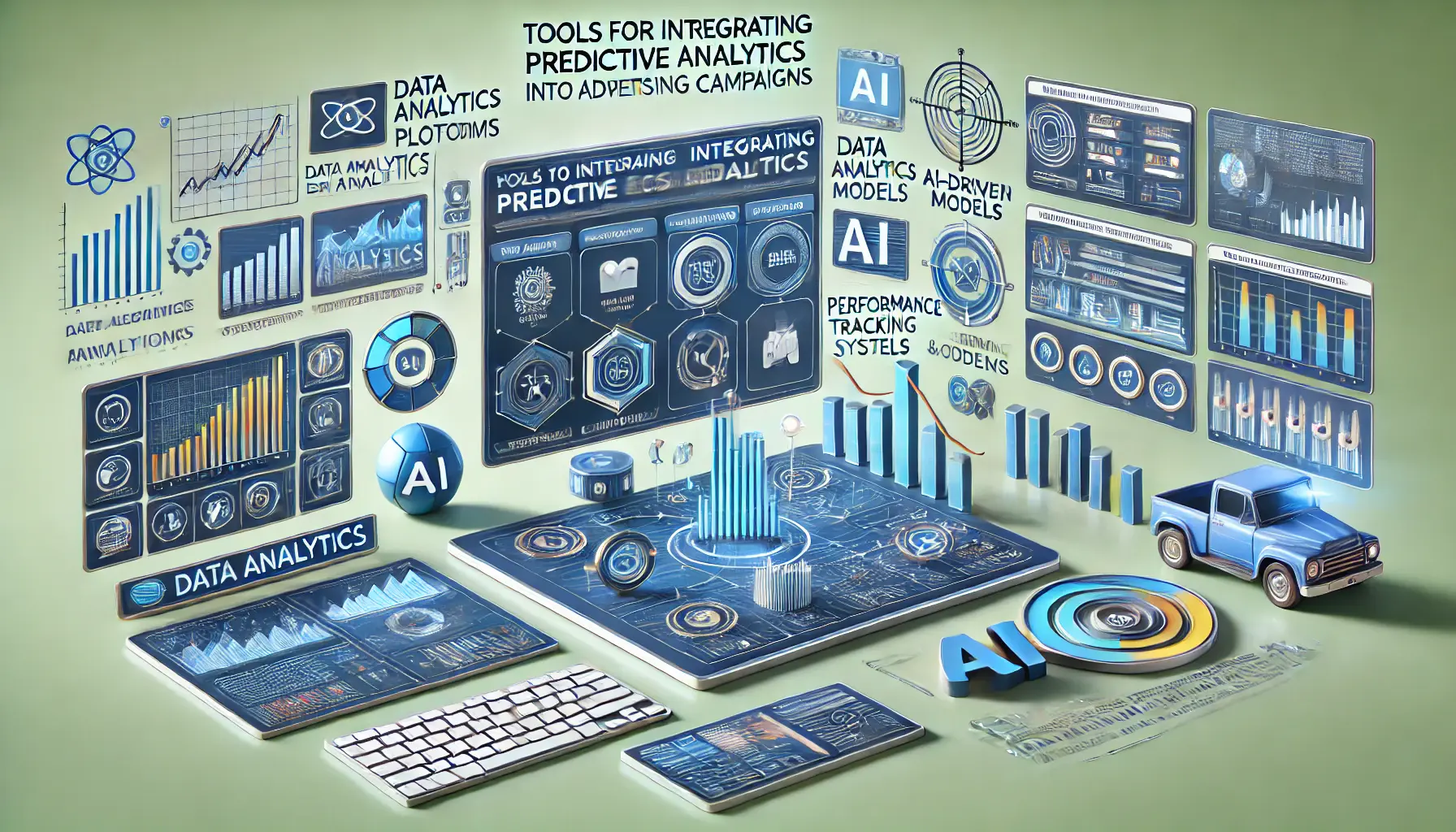
Various tools, such as data analytics platforms and AI models, are used to integrate predictive analytics into advertising campaigns.
Tools for Integrating Predictive Analytics into Campaigns
Several tools are available to help advertisers implement predictive analytics in their campaigns.
Google Analytics, for instance, provides predictive metrics on purchase probability and churn likelihood for each user segment.
Third-party platforms like Mixpanel and Amplitude offer advanced analytics on user behavior.
Many of these tools seamlessly integrate with advertising platforms, enabling advertisers to build predictive models and create targeted ad campaigns based on actionable insights.
By leveraging these tools, advertisers can make data-driven decisions, creating campaigns that connect with users more effectively and ultimately driving higher engagement and better results.
Predictive analytics in AI helps advertisers forecast user behavior, allowing for proactive targeting and improved engagement rates in app advertising.
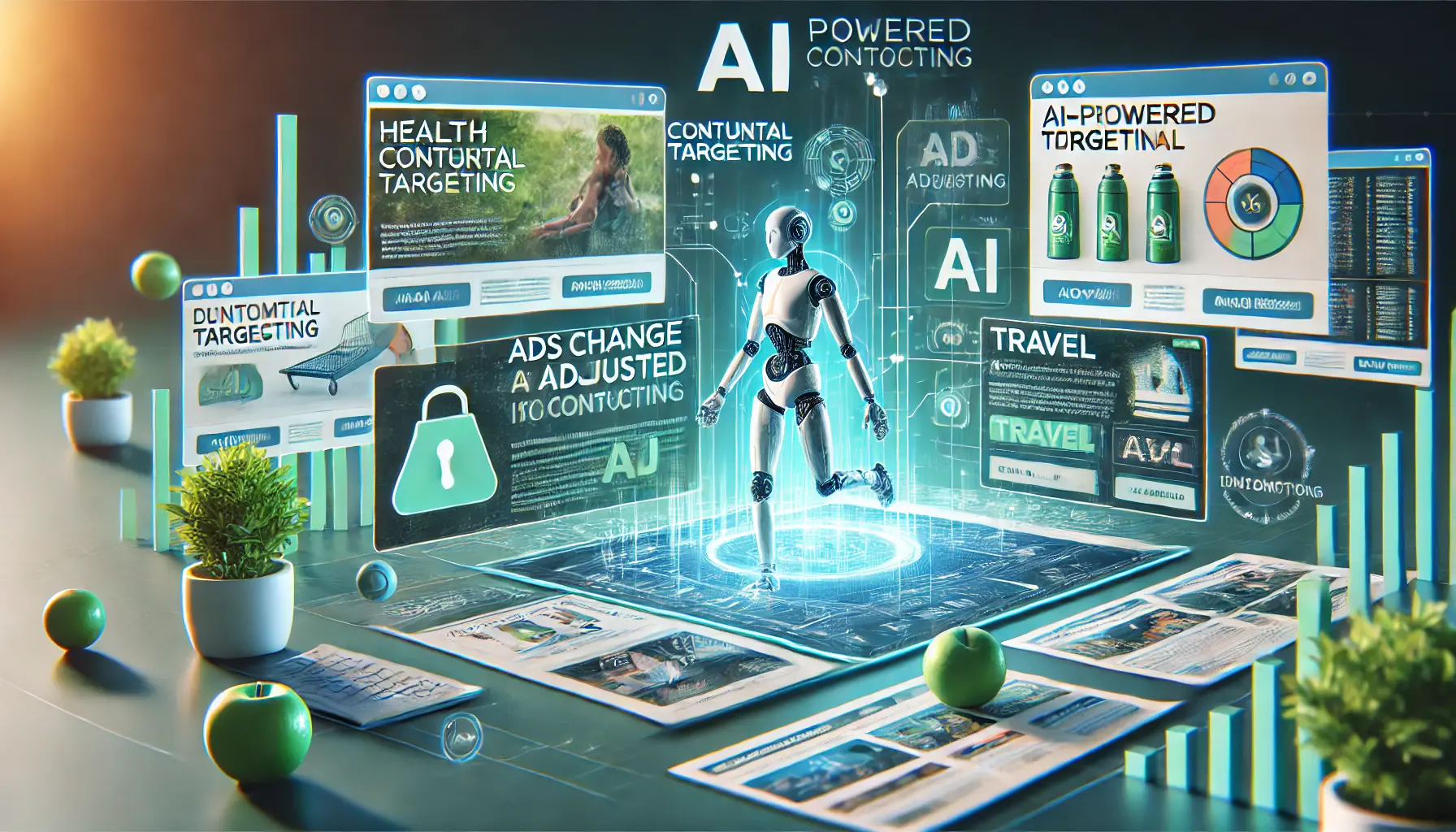
AI-driven contextual targeting optimizes ad relevance by dynamically adjusting content based on the user’s context.
AI-Powered Contextual Targeting: How to Increase the Relevance of Ads?
In the ever-evolving digital advertising environment, AI-powered contextual targeting has become one of the most important strategies for enhancing ad relevance.
By analyzing the content of web pages for context, AI helps advertisers serve ads that perfectly match whatever the user is viewing at that moment.
This approach fosters more meaningful interactions and higher levels of engagement.
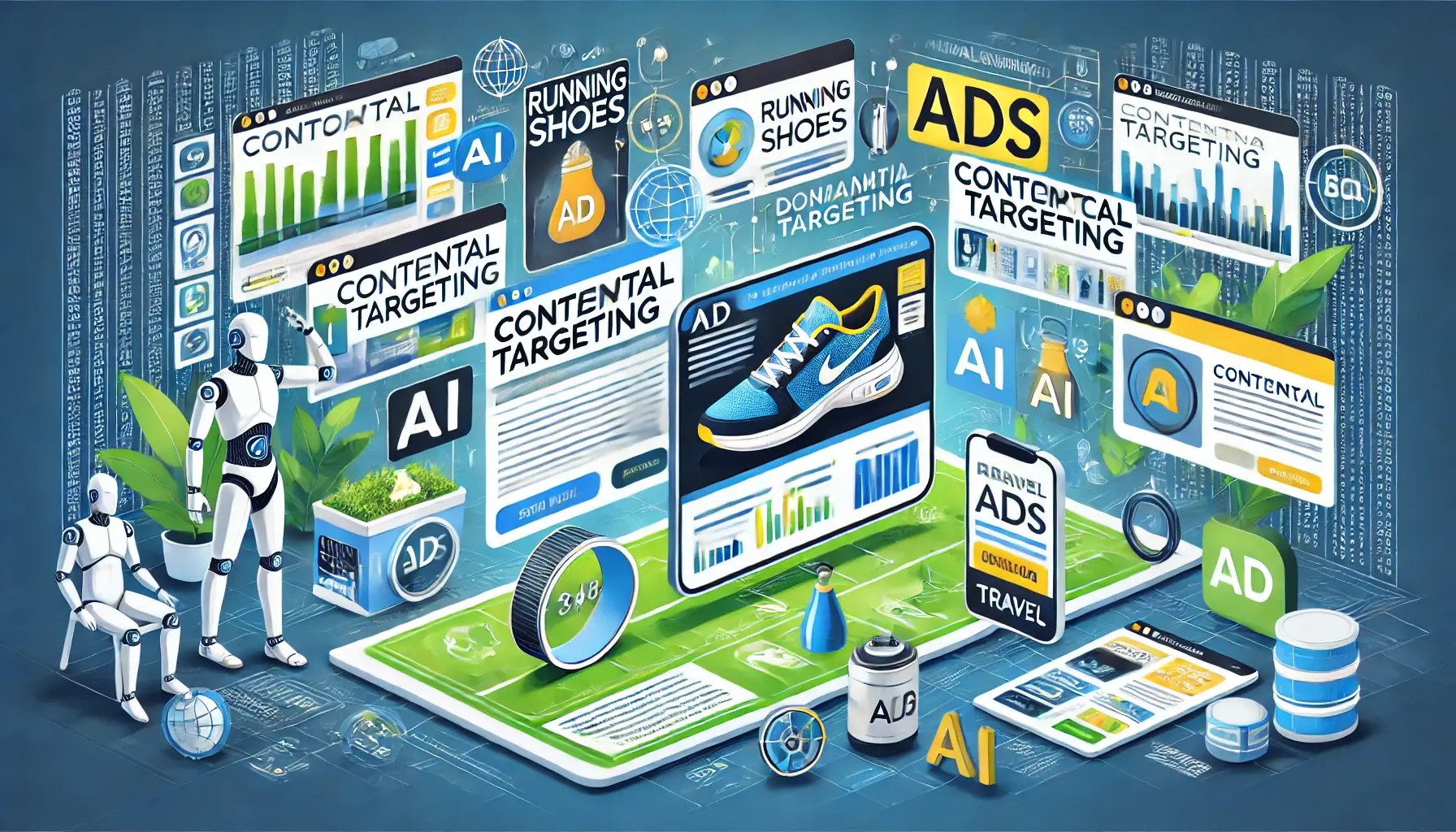
Contextual targeting ensures ads are relevant by dynamically matching them to the content users are engaging with.
Understanding Contextual Targeting
Contextual targeting is a process in which an ad appears on a webpage based on the content rather than user information.
This ensures that ads are relevant to the subject matter being viewed, increasing the likelihood that a user will notice and interact with the ad.
For example, an ad for running shoes displayed on a fitness blog has a much better chance of catching the attention of readers interested in health and exercise.
AI elevates contextual targeting by analyzing web page content more precisely and deeply.
Using natural language processing (NLPNatural Language Processing, an AI branch focused on the interaction between computers and human language) and machine learning algorithms, AI can discern nuances within page content, such as sentiment, tone, and semantics.
This advanced analysis allows ad placements to align more accurately with user interests at that moment.
For instance, AI can differentiate between a web page discussing the benefits of jogging and another covering running injuries, enabling advertisers to reach audiences contextually with ads tailored to the user’s current focus.
This level of sophistication ensures that ads are not only contextually relevant but also sensitive to the user’s mindset.
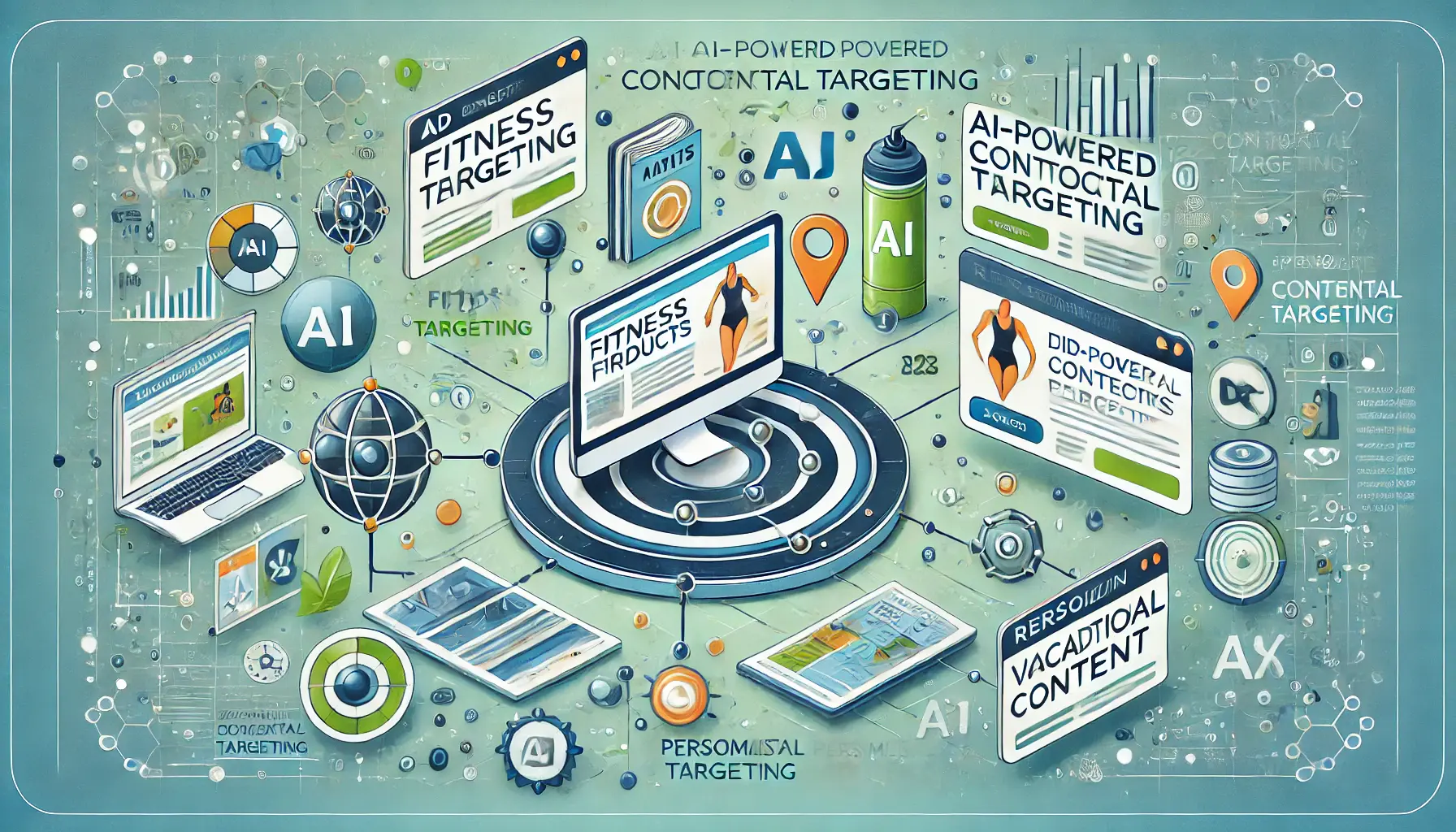
AI-driven contextual targeting enhances ad relevance by delivering personalized content based on user interests.
Benefits of AI-Powered Contextual Targeting
- Increased Relevance: Ads are more likely to resonate with users when they align with the content being viewed, leading to higher engagement rates.
- Privacy Compliance: Contextual targeting does not rely on personal data, making it compliant with privacy regulations and respectful of user anonymity.
- Better Brand Safety: AI ensures ads are placed in appropriate contexts, protecting brand reputation by avoiding placements alongside unsuitable content.
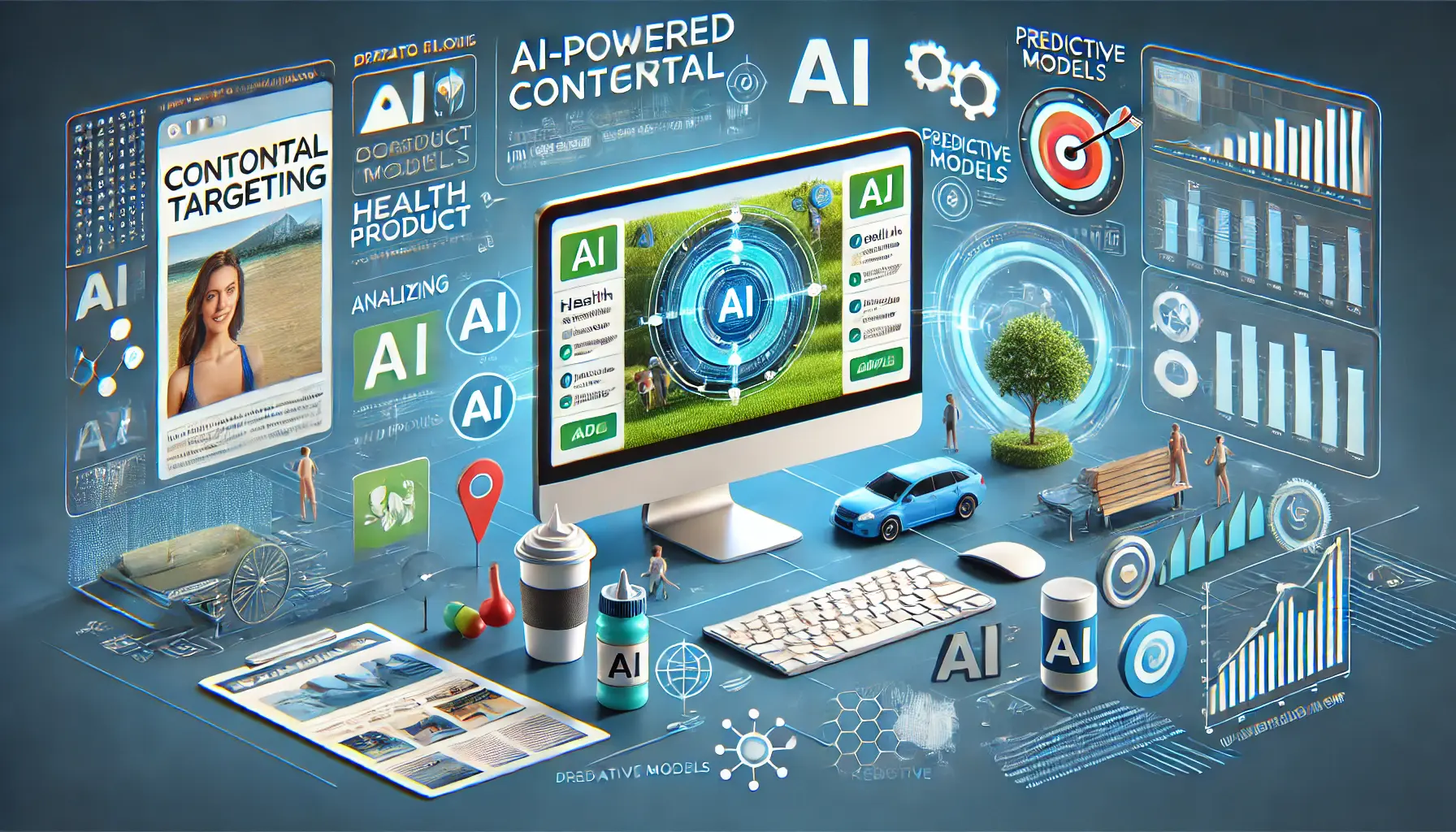
AI-powered contextual targeting is implemented through real-time content analysis, delivering relevant ads to users.
Implementing AI-Powered Contextual Targeting
To effectively implement AI-powered contextual targeting, advertisers should:
- Leverage Advanced AI Tools: Use AI platforms capable of deep content analysis to understand the finer details of web pages.
- Define Contextual Parameters: Establish clear criteria for ad placements, including relevant topics, keywords, and content sentiment.
- Monitor and Optimize: Continuously track ad performance and refine targeting strategies based on real-time data and insights.
By embracing AI-powered contextual targeting, advertisers can create more personalized and effective ad experiences, fostering stronger connections with their audience and driving superior campaign outcomes.
Contextual targeting aligns ads with the content users are viewing, creating more meaningful ad placements and enhancing user engagement.
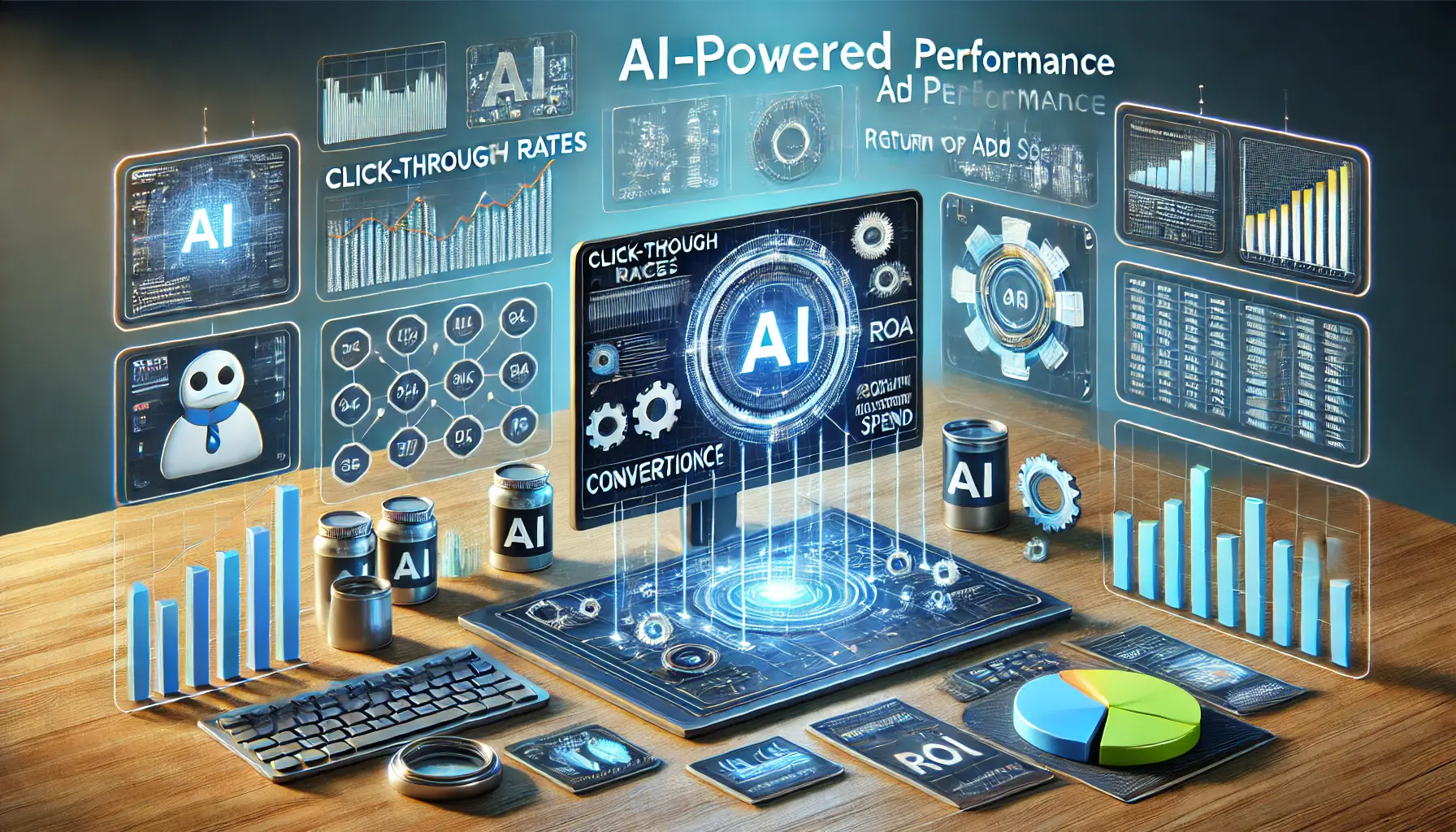
AI-driven ad performance metrics provide actionable insights for optimizing app advertising success.
AI-Powered Ad Performance Metrics: How to Measure App Advertising Success
Understanding your ad performance in the dynamic world of app advertising is crucial.
AI-powered ad performance metrics provide a holistic view of how your ads are performing and enable data-driven decisions to enhance engagement and return on investment (ROI).
With AI, advertisers can dive deeper into performance data, unlocking insights that traditional methods might miss.
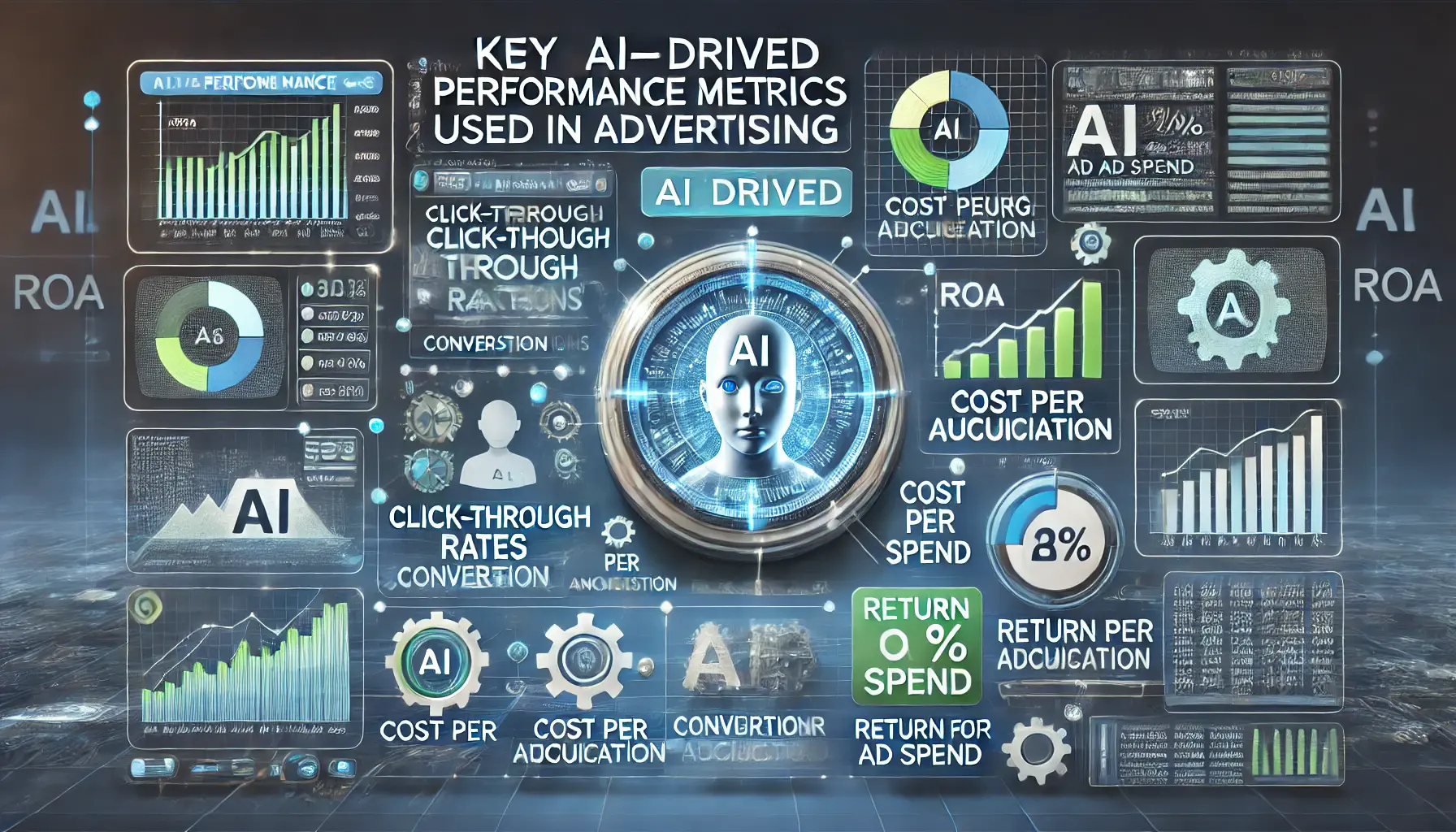
AI-driven performance metrics provide actionable insights to optimize ad campaign effectiveness.
Key AI-Driven Performance Metrics
AI enhances several key advertising performance indicators, including:
- Click-Through Rate (CTR): AI algorithms assess patterns to understand factors that drive higher CTRs, optimizing ad elements to increase user engagement.
- Conversion Rate: AI analyzes user actions and pathways to identify where users drop off, allowing targeted interventions to improve conversions.
- Cost Per Acquisition (CPA): AI optimizes ad spend by identifying the most cost-efficient channels and strategies for acquiring new users.
- Return on Ad Spend (ROAS): Through predictive analytics, AI forecasts potential returns from various ad strategies, aiding budget allocation for maximum ROI.
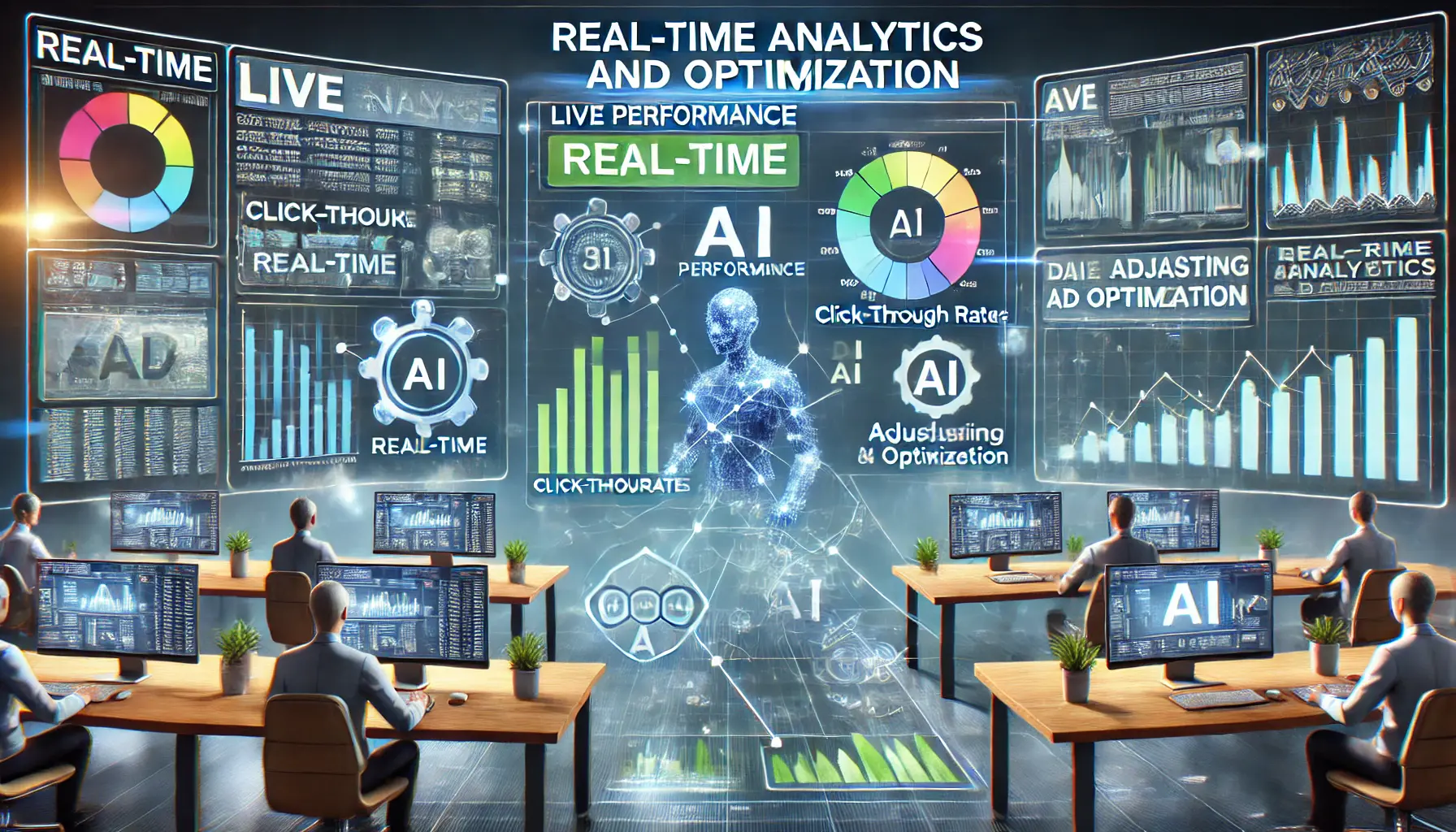
Real-time analytics and optimization enable dynamic adjustments to ad strategies for improved performance.
Real-Time Analytics and Optimization
One of the significant advantages of AI in ad performance measurement is real-time analyticsData analysis that occurs instantly or within a very short time after data is generated.
AI systems monitor ad campaigns continuously and generate immediate feedback on their effectiveness.
This real-time information allows advertisers to:
- Immediately Identify Poorly Performing Ads: Quick identification enables prompt adjustments to ad content or targeting strategies.
- Optimize Budget Usage: Resources can be redirected to high-performing ads or channels, ensuring efficient use of resources.
- Improve User Targeting: AI refines audience segments based on real-time engagement data, resulting in better ad relevance and effectiveness.
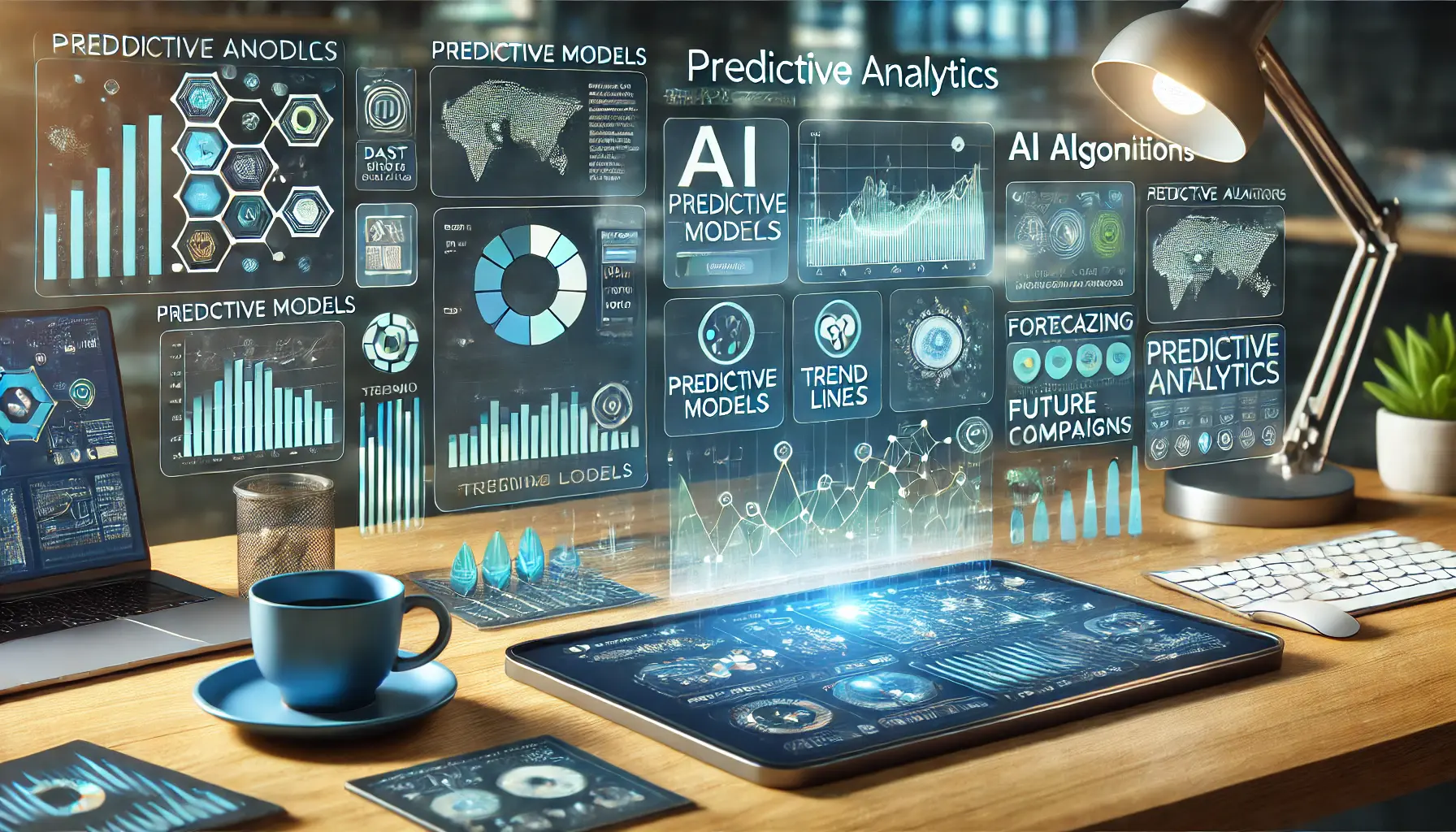
Predictive analytics enables data-driven insights for planning and optimizing future advertising campaigns.
Predictive Analytics for Planning Future Campaigns
Beyond analyzing current performance, AI uses predictive analytics to estimate outcomes for future campaigns.
By analyzing historical data and identifying trends, AI models can:
- Foresee User Behavior: Predict which user segments are most likely to engage or convert, allowing proactive targeting.
- Inform Creative Strategies: Provide insights into which ad creatives are likely to resonate with the target audience.
- Guide Budget Planning: Offer projections on potential ROI for various campaign strategies, supporting better decision-making.
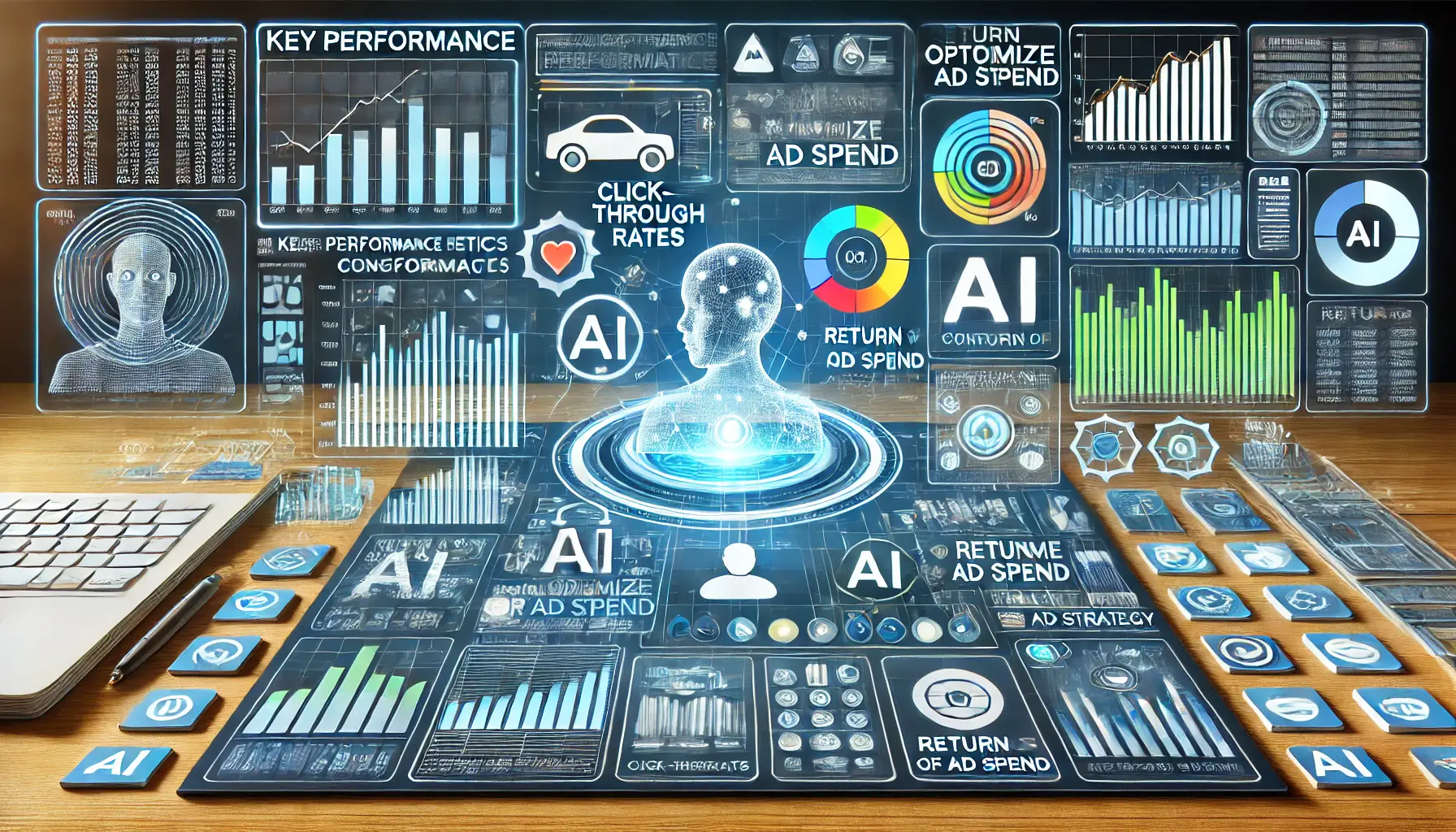
AI-driven metrics enable advertisers to optimize their strategies by analyzing key performance indicators in real-time.
Applying AI-Driven Metrics in Your Strategy
To effectively apply AI-driven ad performance metrics in your app advertising strategy:
- Apply AI Analytics Tools: Use platforms that offer AI-powered analytics to gain deeper insights into ad performance.
- Train Your Team: Ensure your marketing team understands how to interpret AI-driven data and apply insights to campaign strategies.
- Monitor and Adapt Continuously: Regularly review performance data and be prepared to adjust strategies based on AI-generated insights.
Embracing AI-driven ad performance metrics provides advertisers with a more granular view of their campaigns, enabling more effective strategies and yielding improved outcomes in app advertising.
AI-driven ad performance metrics provide real-time insights, allowing advertisers to optimize campaigns for engagement, conversions, and efficient ad spend.
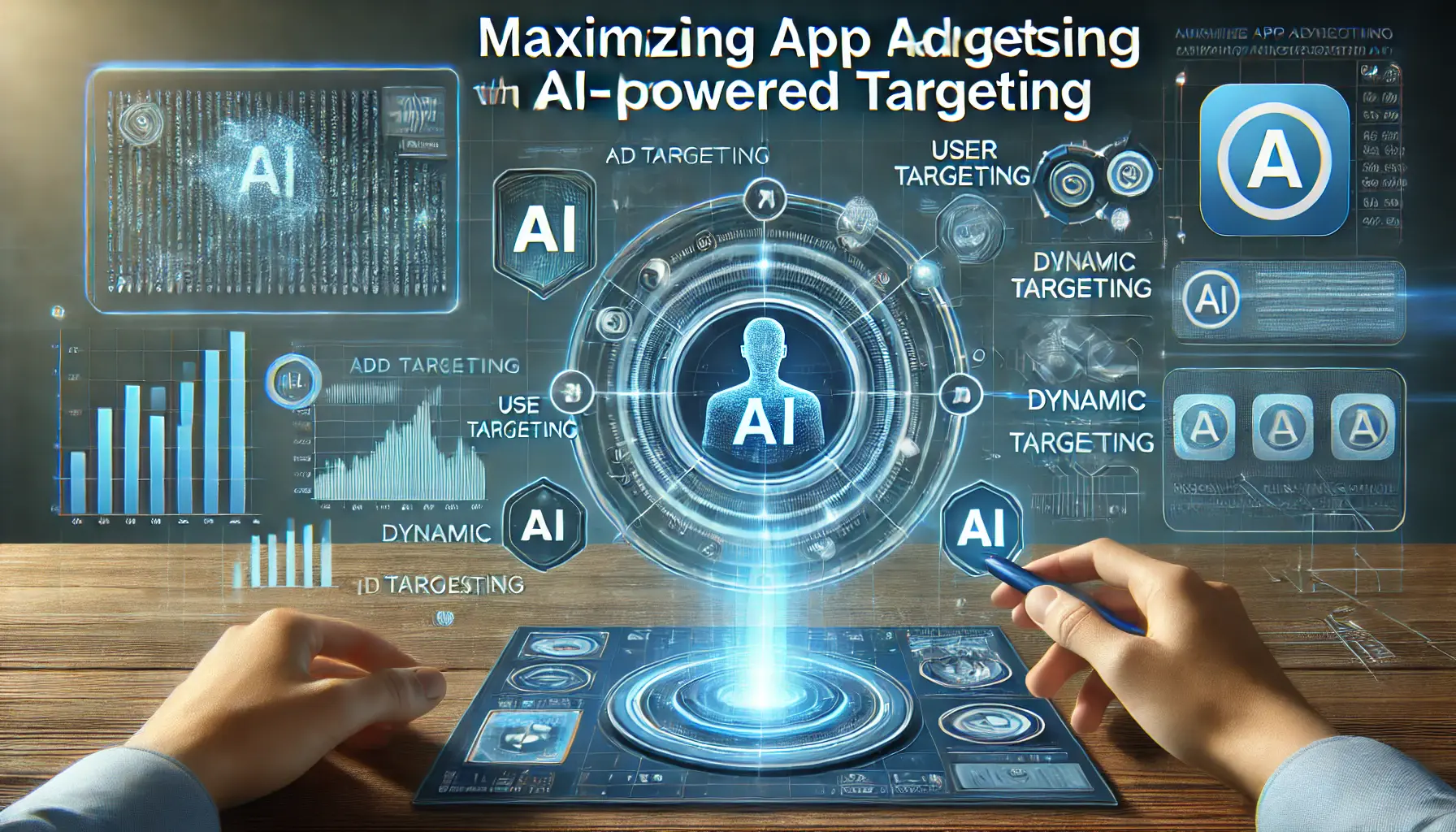
AI-powered targeting maximizes app advertising effectiveness by refining strategies through real-time data analysis.
Maximizing App Advertising with AI-Powered Targeting
App advertising continues to evolve, and AI-powered targeting has become an invaluable tool for reaching audiences more effectively and efficiently.
By placing artificial intelligence at the core of ad-targeting strategies, advertisers can make data-driven decisions that amplify engagement and maximize return on investment (ROI).
AI enhances personalization, predicts user behaviors, and optimizes real-time targeting, revolutionizing how app ads connect with users.
Building personalized audience profiles, making real-time adjustments, and leveraging predictive insights are essential steps in the AI-powered targeting process that create more meaningful and relevant advertising experiences.
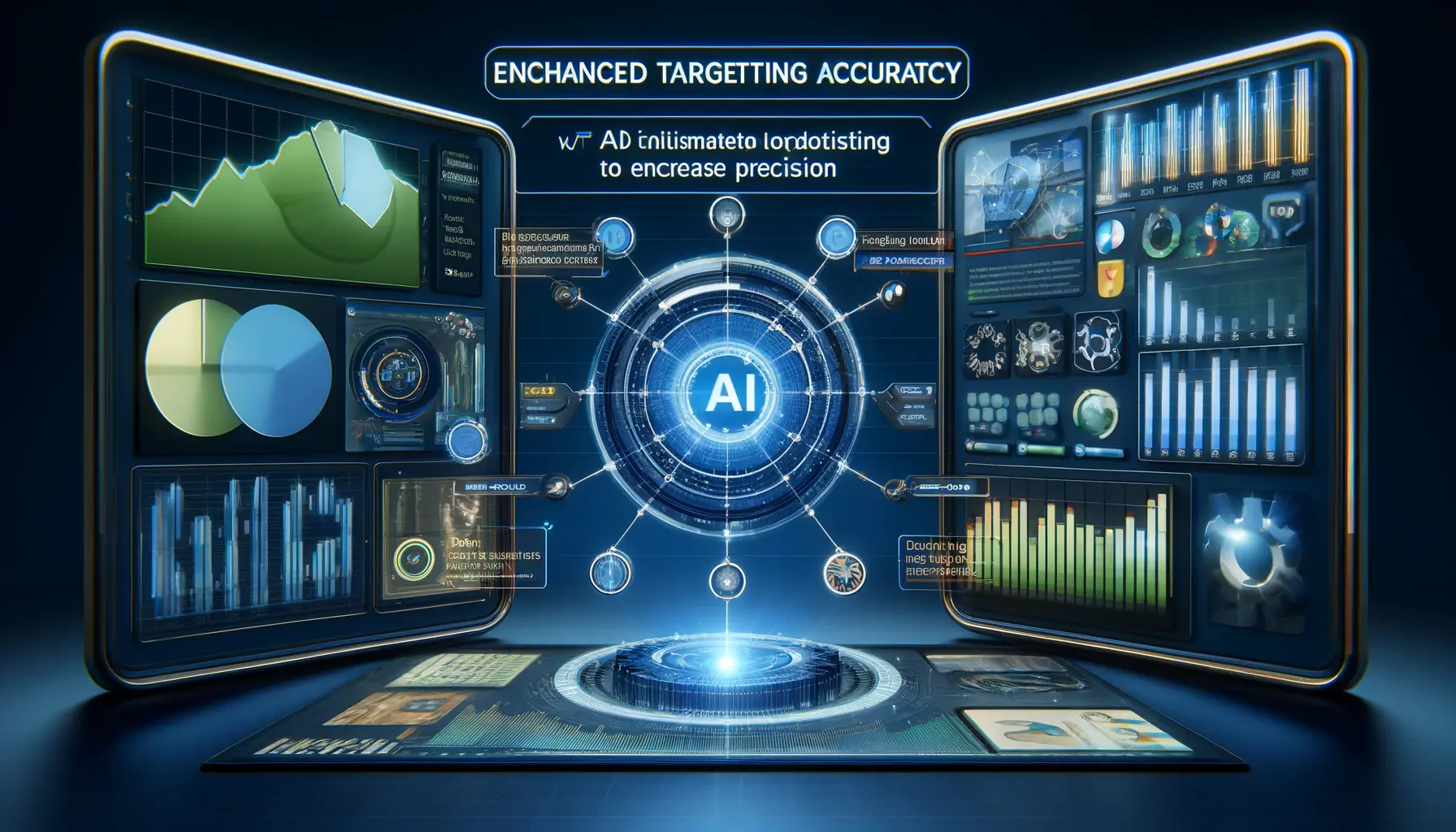
AI enhances targeting accuracy by adjusting ad strategies in real-time based on user data and behavior insights.
Enhancing Targeting Accuracy with AI
AI-driven targeting allows advertisers to dive deeper into audience data, segmenting users based on behaviors, interests, and context.
By identifying patterns and analyzing user data in real time, AI refines targeting accuracy, helping advertisers place ads at the right time and place for maximum impact.
Personalized targeting ensures ads resonate with specific user groups, a critical factor in driving higher engagement rates.
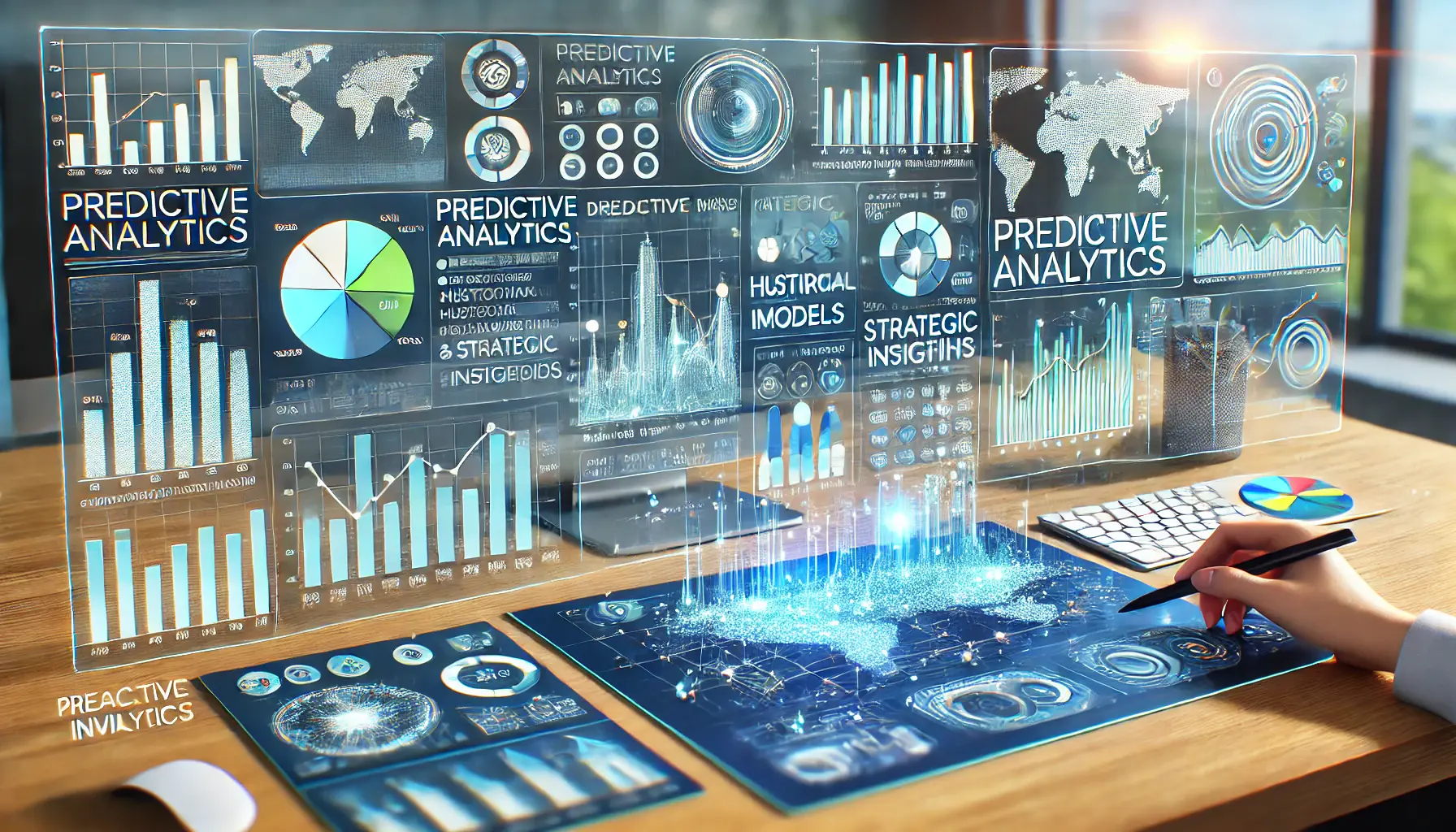
Predictive analytics provide valuable strategic insights for shaping effective advertising campaigns.
Leveraging Predictive Analytics for Strategic Insights
Predictive analytics enables advertisers to forecast user behaviors and plan campaigns effectively.
By accurately predicting user actions—such as app installs or in-app purchases—advertisers can proactively create strategies to drive engagement and loyalty.
AI-driven predictive models highlight high-value audience segments and help optimize ad placements, ensuring effective ad spend that maximizes ROI.
Predictive analytics not only enhances the efficiency of current campaigns but also provides a clear roadmap for future advertising, aligning resources with opportunities for optimal returns.
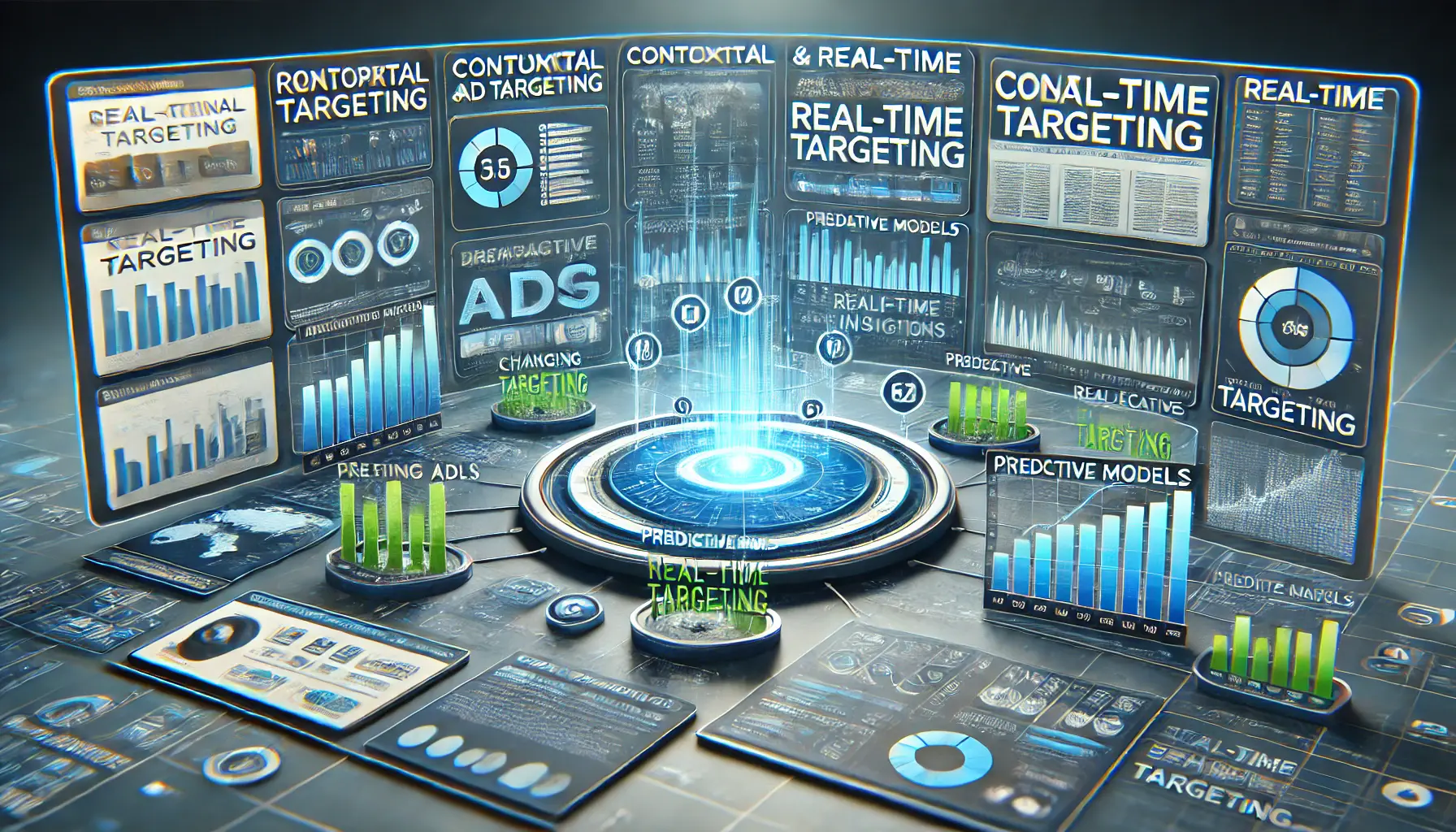
Contextual and real-time targeting dynamically adjusts ad strategies to deliver personalized, relevant content to users.
Implementing Contextual and Real-Time Targeting
AI-powered contextual targeting boosts ad relevance by aligning ads with the content users are currently viewing, significantly increasing engagement.
This approach respects user privacy while ensuring ads are displayed in suitable environments related to user interests.
Real-time targeting takes this a step further by allowing advertisers to monitor ad performance instantaneously and make adjustments as needed.
By continuously adapting campaigns based on real-time data, advertisers keep their ads relevant and capitalize on ever-evolving user trends.
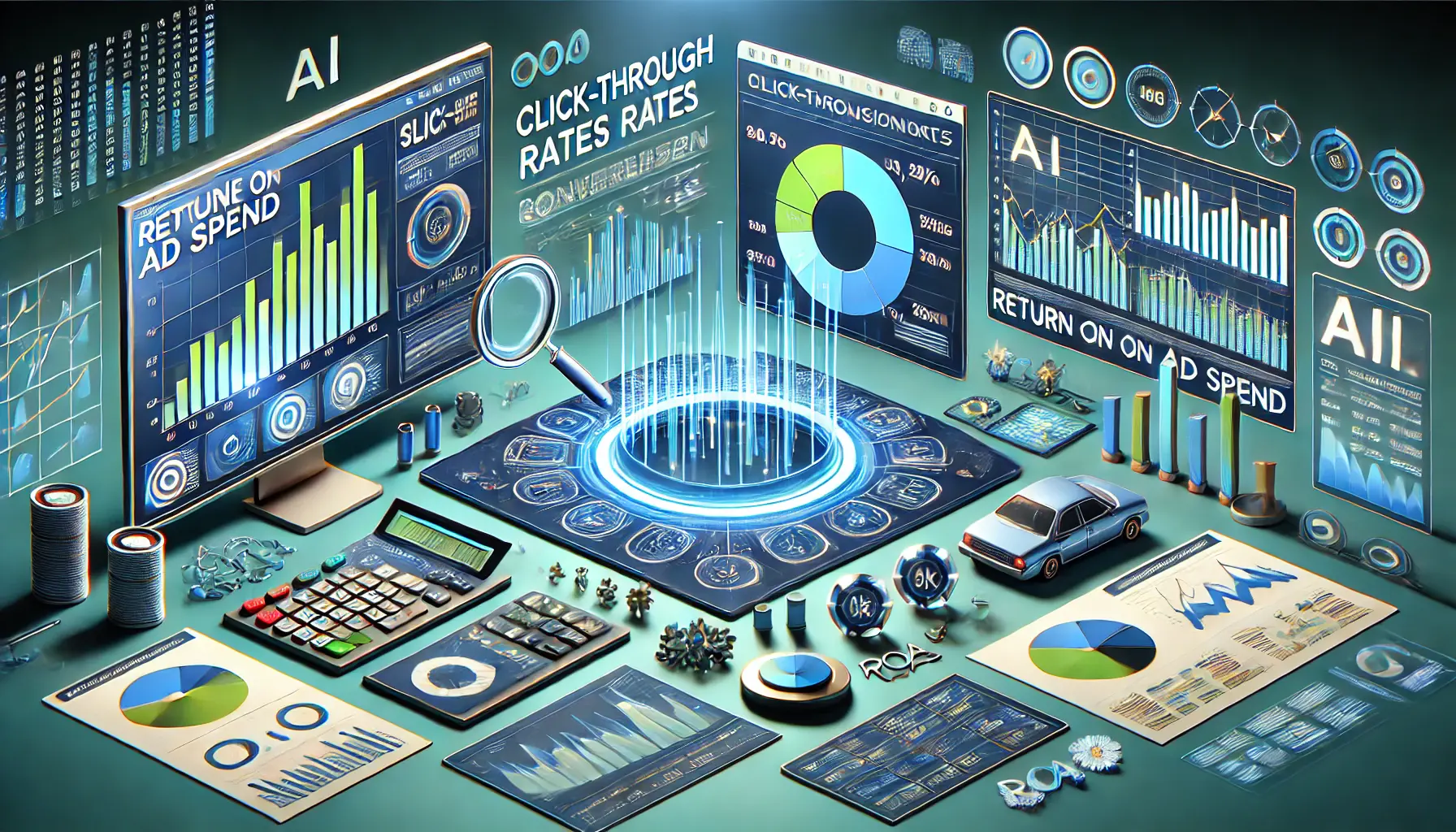
AI-driven metrics provide valuable insights into advertising success, optimizing campaign performance.
Measuring Success with AI-Driven Metrics
Accurate measurement is essential for any successful advertising strategy, and AI-driven metrics offer deep insights into ad performance.
Core metrics such as CTRClick-Through Rate, the percentage of users who click on an ad or link out of total views, conversion rateThe percentage of users who take a desired action, such as making a purchase, and ROASReturn on Ad Spend, a metric measuring revenue earned for each dollar spent on advertising become even more powerful with AI, which reveals the factors driving these numbers and optimizes them accordingly.
Real-time analytics allow advertisers to promptly identify underperforming ads, enabling swift adjustments to maintain campaign efficiency and effectiveness.
AI-driven performance metrics empower advertisers to continually refine their strategies, achieving superior results in app advertising.
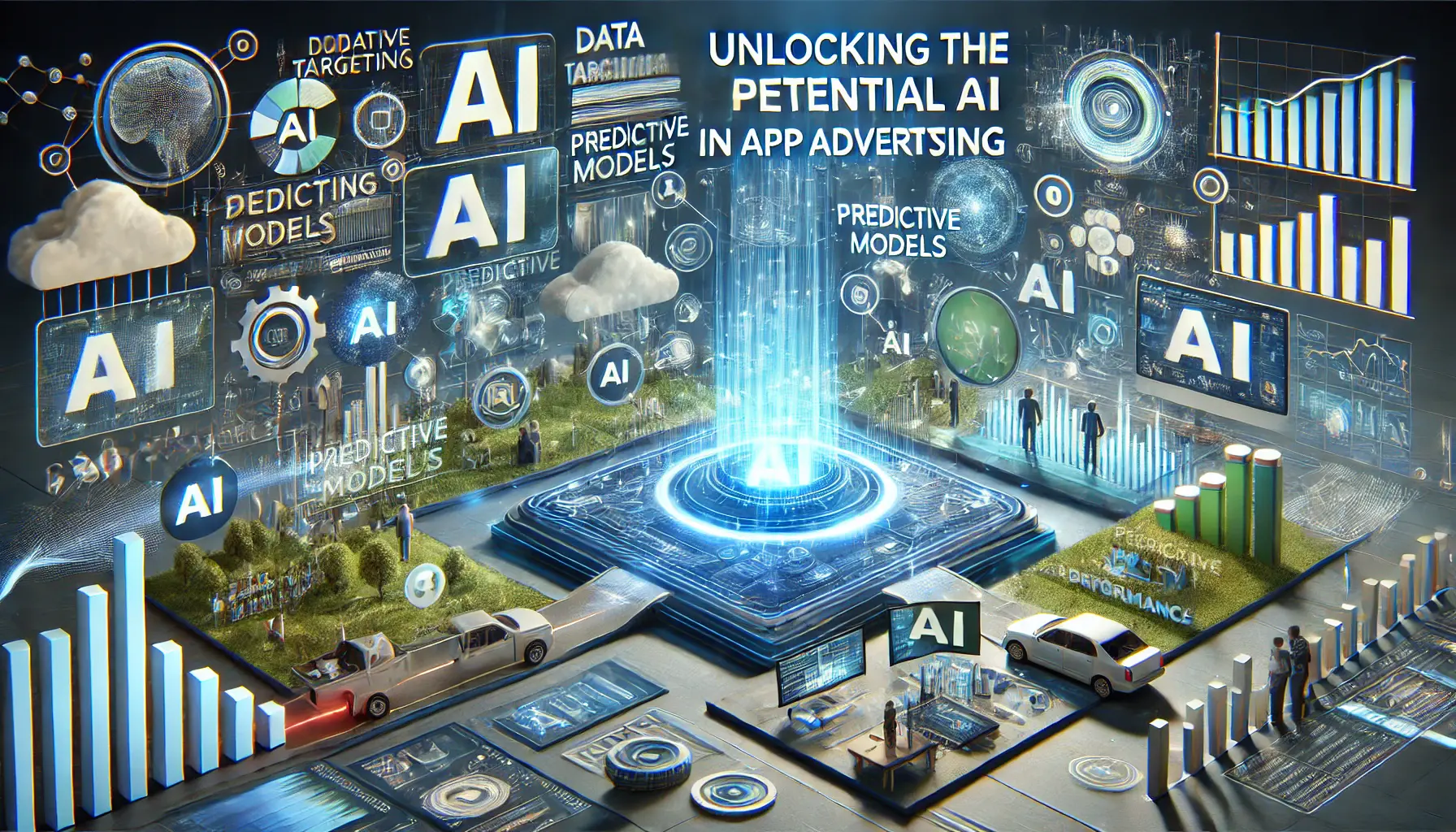
AI-driven optimization unlocks the full potential of app advertising, enhancing targeting and user engagement.
Conclusion: Unlocking the Potential of AI in App Advertising
AI-powered targeting is transforming app advertising by making ads more relevant, engaging, and strategically aligned with user needs.
As the digital landscape becomes increasingly competitive, the capabilities of AI in personalization, prediction, and optimization become indispensable assets for advertisers looking to maximize their campaign impact.
With AI-driven audience profiles, predictive insights, contextual relevance, and real-time performance metrics, advertisers can connect with their audiences on a deeper level.
Embracing AI-powered targeting allows advertisers to build campaigns that not only reach the right users but do so in a natural and engaging way.
By unlocking the full potential of AI, app advertisers can achieve a new level of efficiency and effectiveness, resulting in higher engagement, improved conversion rates, and a significantly enhanced ROI.
AI-powered targeting in app ads enhances audience relevance, engagement, and campaign ROI by combining personalization, predictive analytics, and contextual targeting.
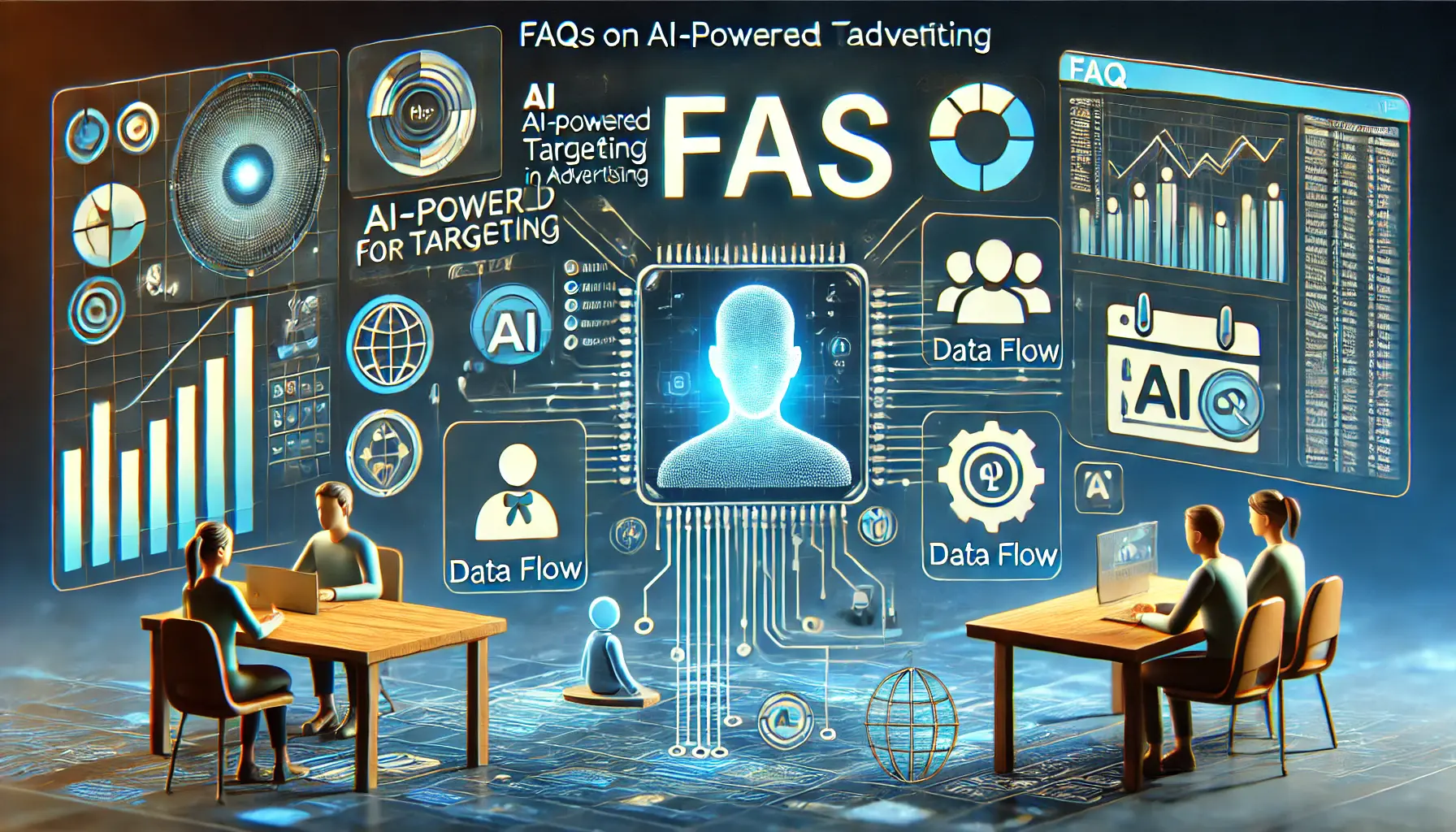
AI-driven targeting provides valuable insights for app advertising, answering common questions about its effectiveness.
Your campaigns can be managed by an agency specialized in Google Ads, check out our service page.
FAQs on AI-Powered Targeting for App Advertising
With AI gaining momentum in the world of app advertising, many questions arise regarding its implementation and benefits.
Below are common queries with concise answers to help you better understand AI-powered targeting.
AI-powered targeting uses artificial intelligence to analyze user data, helping advertisers deliver more personalized and relevant ads within apps to increase user engagement and campaign effectiveness.
AI processes vast datasets to identify patterns and user behaviors, enabling precise audience segmentation and delivering ads that align closely with each user’s interests and preferences.
Yes, AI uses predictive analytics to forecast user actions, such as app installations or in-app purchases, allowing advertisers to create campaigns that meet user needs effectively.
AI-driven contextual targeting places ads in environments that match the content users are engaging with, increasing ad relevance, enhancing user experience, and boosting overall engagement rates.
AI provides real-time analytics on key performance indicators, such as click-through and conversion rates, offering insights that help advertisers optimize campaigns for better results.
Yes, AI-powered targeting can be designed to comply with privacy laws by focusing on contextual and anonymized data, balancing user privacy with delivering relevant ads.
Small businesses can use AI-powered advertising platforms with accessible audience targeting tools and predictive analytics to measure campaign performance effectively with limited resources.
Challenges include ensuring data privacy, managing the complexity of AI systems, and requiring continuous monitoring to maintain accuracy and relevance in dynamic user environments.
AI analyzes performance data to allocate budgets efficiently, focusing on high-performing segments and strategies, which maximizes ROI and reduces unnecessary expenditures.