The world of digital marketing is ever-changing, and one must change with the times.
Currently, one of the most innovative tools in advertising is machine learning.
This sets the bar high where ad creation, targeting, and optimization are concerned.
For app developers and marketers, the big difference would be between an ad campaign that reaches incredible heights and one that completely flops.
But what makes machine learning so powerful, and how can you use it effectively in your app advertising strategy?
Let’s dive into the techniques that can elevate your campaigns and maximize their impact.
- Understanding Machine Learning in App Advertising
- Technique 1: Predictive Targeting for Enhanced User Engagement
- Technique 2: Dynamic Ad Personalization Using Machine Learning
- Technique 3: Optimizing Ad Spend with Automated Bidding Strategies
- Implementing Machine Learning in Your App Advertising Strategy
- Conclusion: Unlocking the Potential of Machine Learning in App Advertising
- FAQs about Machine Learning in App Advertising
Understanding Machine Learning in App Advertising
Machine learning is not just a buzzword; it’s a game-changer for app advertising.
Through algorithms driven by data, machine learning can help advertisers predict user behavior, personalize content, and optimize campaigns in real time.
This equates to more relevant ads, users better engaging with the ads, and higher ROIReturn on Investment, a measure of the profitability of an investment..
Imagine being able to predict what your audience wants before they do.
That’s what machine learning can bring to a business.
It turns vast amounts of data into actionable insights, allowing you to connect with users on a deeper level.
But what exactly is machine learning, and how does it transform app advertising?
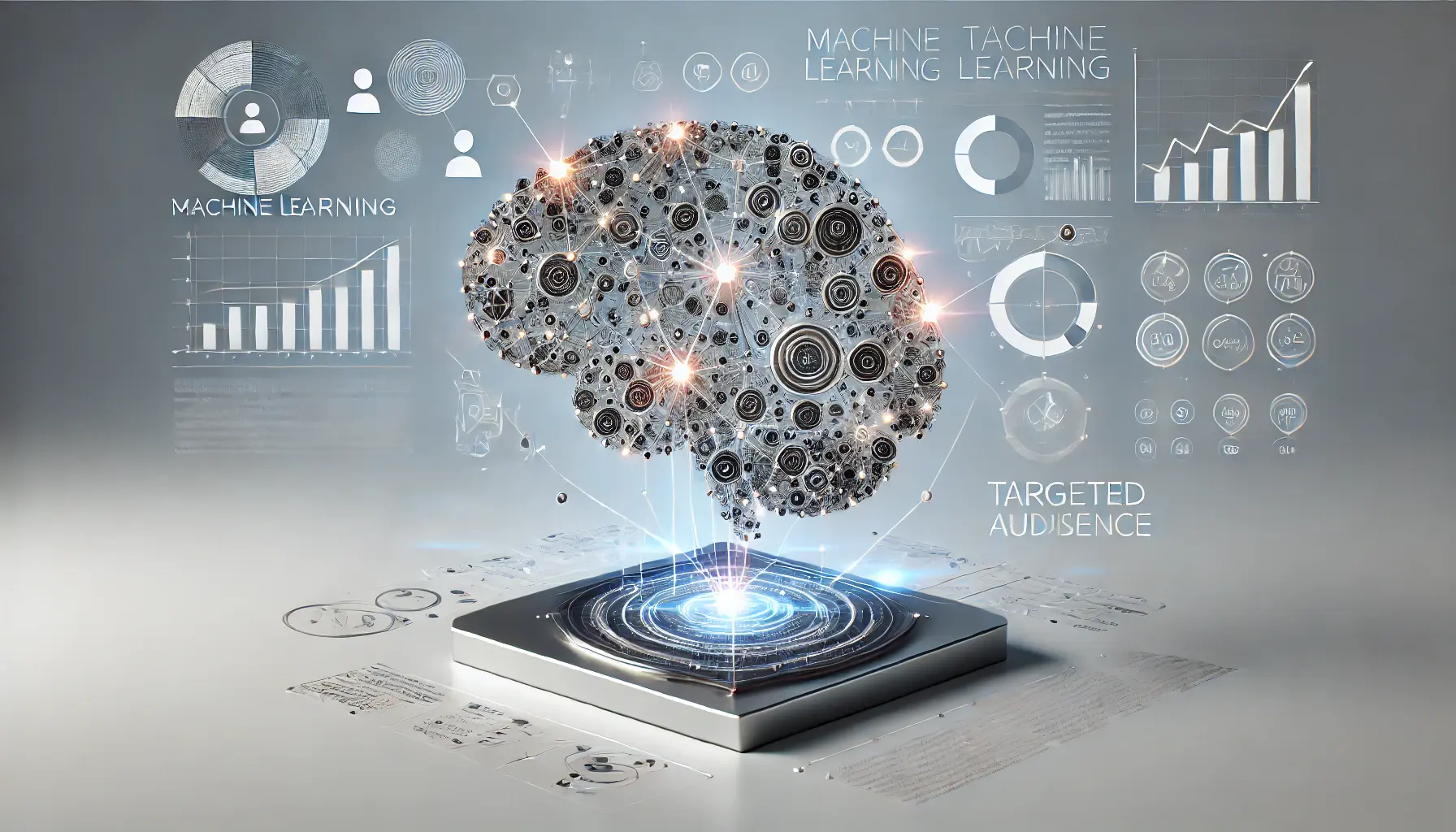
Illustrating the fusion of machine learning and advertising through data-driven insights and precision.
Defining Machine Learning and Its Role in Advertising
Machine learning is a subset of artificial intelligence (AIArtificial Intelligence, the simulation of human intelligence by machines.) that enables systems to learn and improve from experience without being explicitly programmed.
Precisely, in advertising, it comprises user data analysis for pattern identification to predict future behavior.
This capability helps marketers create highly targeted and effective ad campaigns.
- Data Analysis: Machine learning algorithms sift through massive amounts of data to find trends and insights.
- Predictive Modeling: These insights are used to forecast user preferences and behaviors.
- Automation: Machine learning automates decision-making processes, ensuring ads are delivered at the right time to the right audience.
By integrating machine learning into your app advertising strategy, you can achieve unparalleled precision and efficiency.
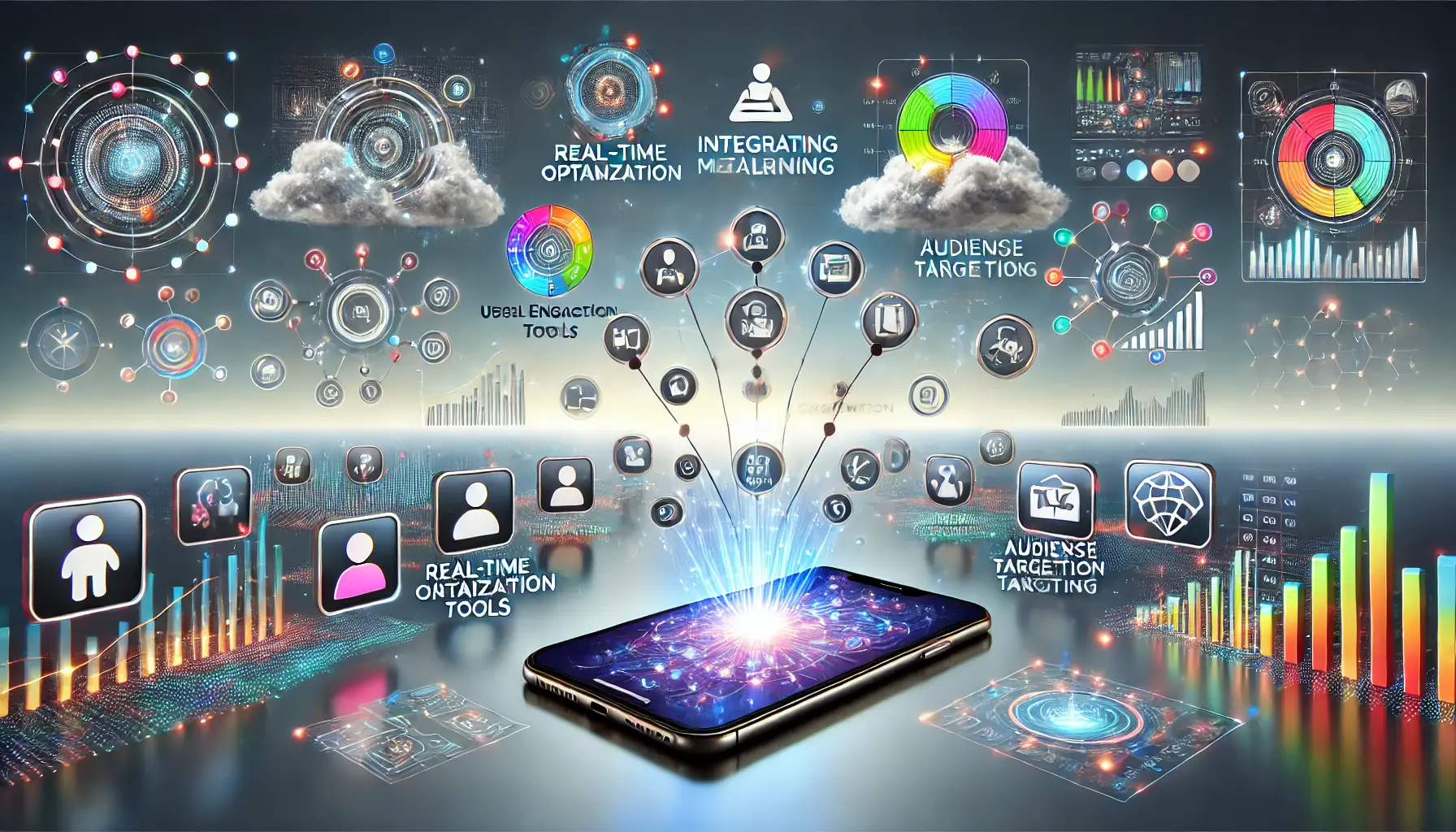
Illustrating the advantages of using machine learning for enhanced targeting, real-time optimization, and personalized app ads.
Key Benefits of Integrating Machine Learning into App Ads
What makes machine learning indispensable for app advertising?
Here are some of the highlighted benefits:
- Enhanced Targeting: Reach better audience segments with your ad targeting, based on their behaviors and preferences.
- Improved ROI: Optimize your ad spend by focusing on high-value users who are more likely to convert.
- Personalized Content: Tailor your ads to individual users, raising engagement and satisfaction.
- Real-Time Optimization: Adapt your campaigns on the fly for the best possible performance.
Integrating machine learning into your app advertising is not about keeping abreast of trends but setting new standards.
With the right approach, you can harness this technology to drive exceptional results for your campaigns.
Machine learning transforms vast data into actionable insights, enabling more personalized and efficient advertising strategies.
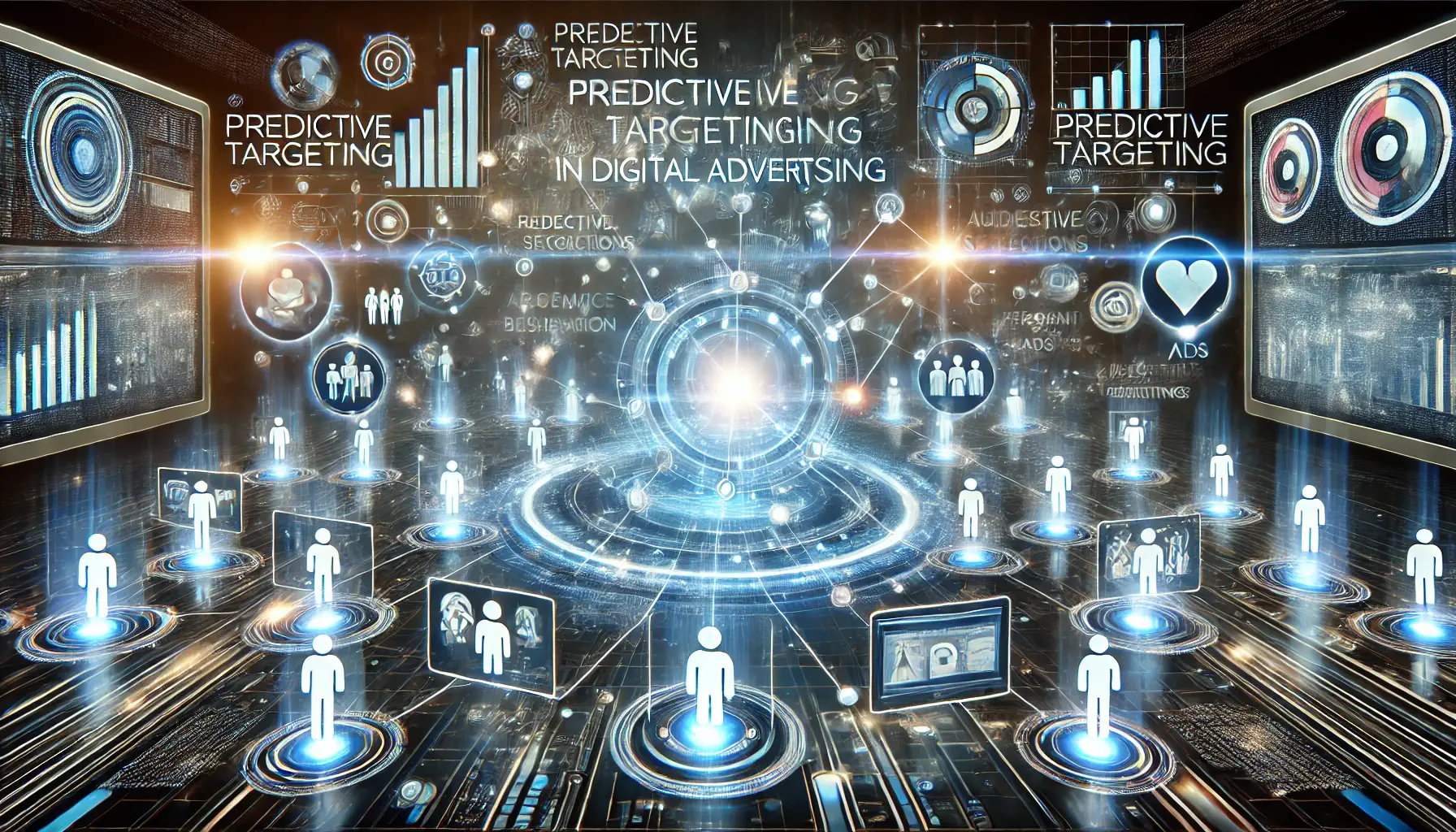
Visualizing how predictive targeting uses data and algorithms to enhance user engagement through personalized content.
Technique 1: Predictive Targeting for Enhanced User Engagement
In the competitive landscape of app advertising, reaching the right audience is paramount.
Predictive targeting, powered by machine learning, enables advertisers to anticipate user behaviors and preferences, ensuring that ads resonate with potential users.
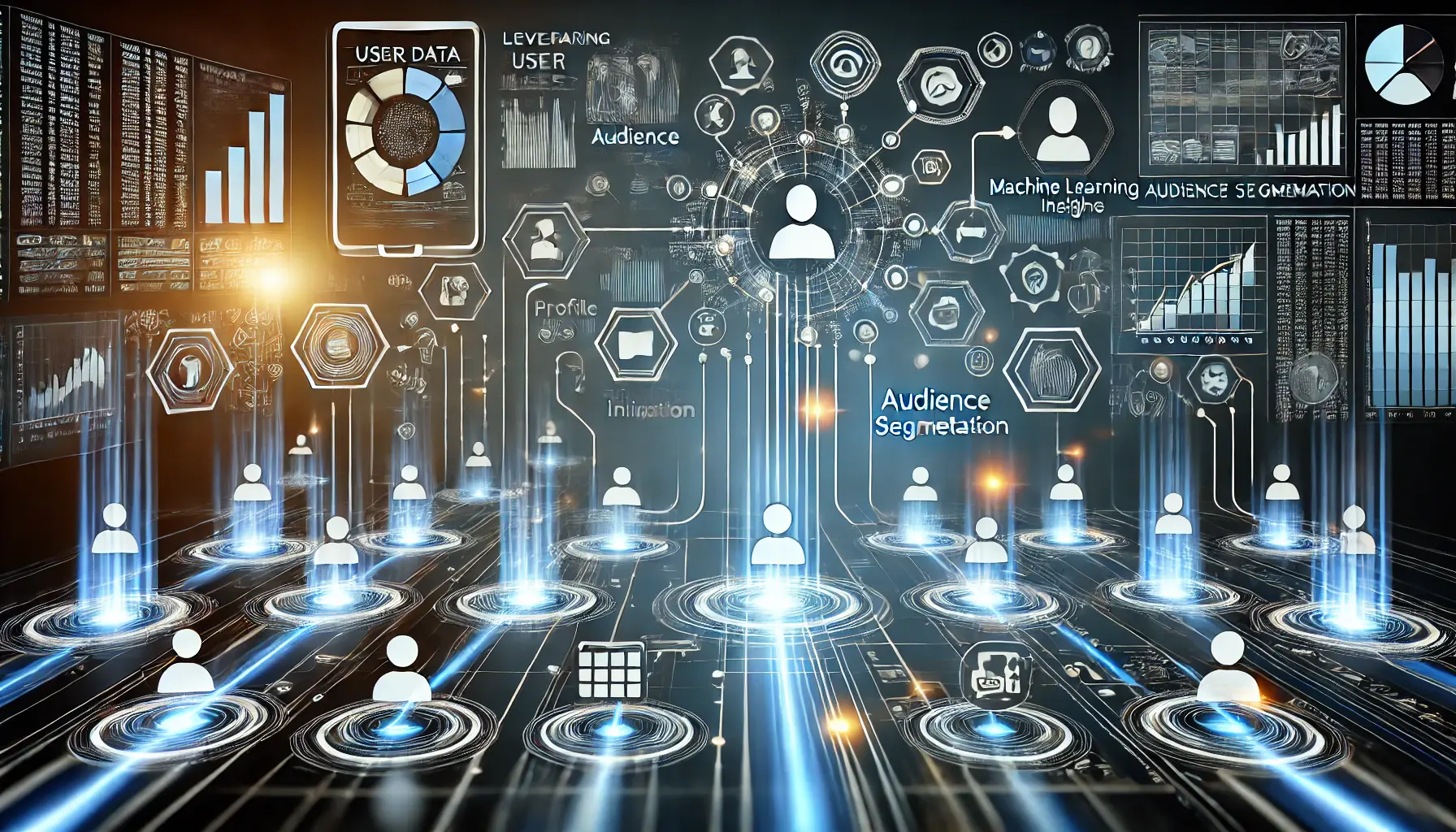
Illustrating how user data is leveraged for audience segmentation, creating targeted and personalized digital marketing strategies.
Leveraging User Data for Accurate Audience Segmentation
Machine learning algorithms analyze vast amounts of user data, including browsing history, in-app behaviors, and demographicStatistical data relating to populations, such as age, gender, or income. information.
By identifying patterns in this data, advertisers can more accurately segment audiences.
This segmentation allows for the delivery of tailored ads that align closely with each group’s interests and needs.
- Behavioral Analysis: Understanding user interactions to predict future actions.
- Demographic Profiling: Categorizing users based on age, location, and other demographic factors.
- Interest Mapping: Identifying user interests to align ad content accordingly.
Implementing predictive targeting enhances the relevance of ads, leading to higher engagement rates and improved return on investment (ROI).
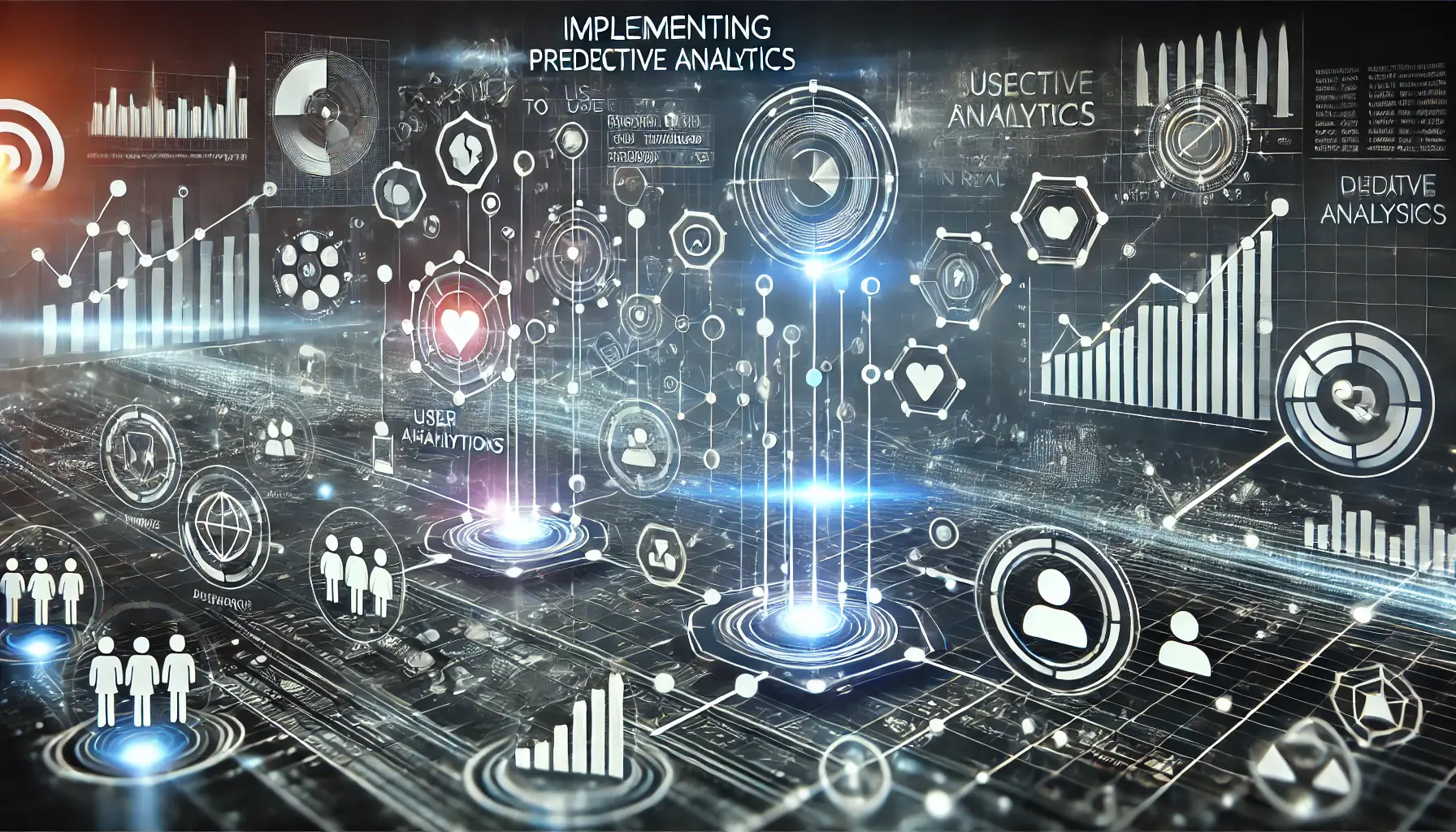
Visualizing the power of predictive analytics in forecasting user behavior through data flow and trend analysis.
Implementing Predictive Analytics to Anticipate User Behavior
Predictive analytics utilizes historical data to forecast future user actions.
In app advertising, this involves identifying users who are most likely to install an app, make in-app purchases, or engage with specific content.
By focusing on these high-potential users, advertisers can distribute resources more effectively.
- Conversion Probability: Estimating user conversion likelihood based on past behaviors.
- Churn Prediction: Detecting the risk of user disengagement and enabling retention strategies.
- Lifetime Value Estimation: Anticipating the lifetime value of users to prioritize high-value segments.
Ultimately, using predictive analyticsThe use of data, algorithms, and machine learning to forecast future outcomes. in your advertising method allows you to anticipate the needs and preferences of your users and build better engagement with them.
- Predictive targeting anticipates user behavior to ensure ads resonate with potential users.
- Segment audiences based on patterns for tailored ad delivery.
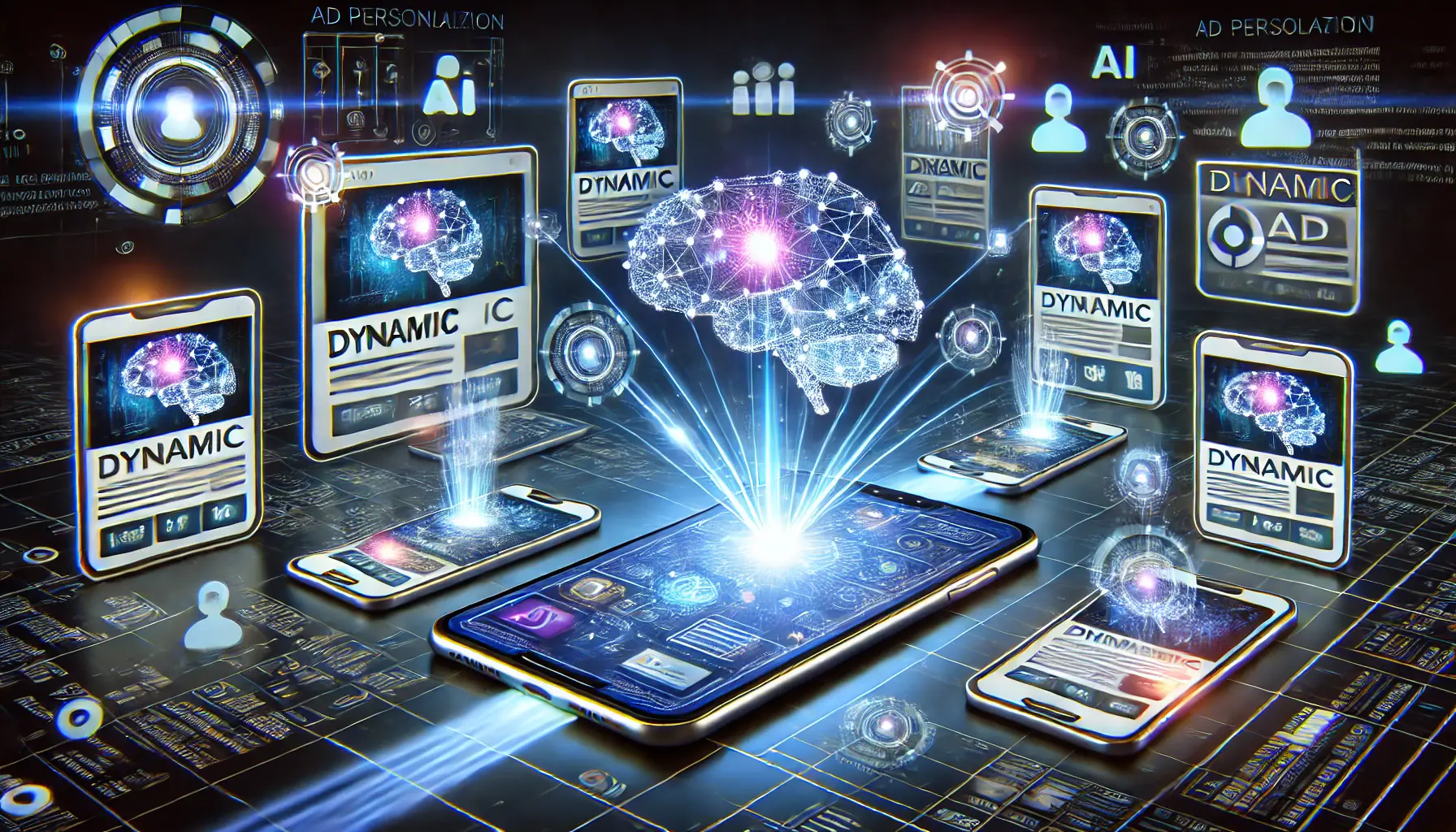
Visualizing how machine learning enables dynamic personalization of ads in real-time, enhancing user engagement.
Technique 2: Dynamic Ad Personalization Using Machine Learning
In today’s digital landscape, users are inundated with advertisements, making it crucial for app marketers to stand out.
Dynamic ad personalization, powered by machine learning, enables the creation of tailored ad experiences that resonate with individual users, thereby enhancing engagement and conversion rates.
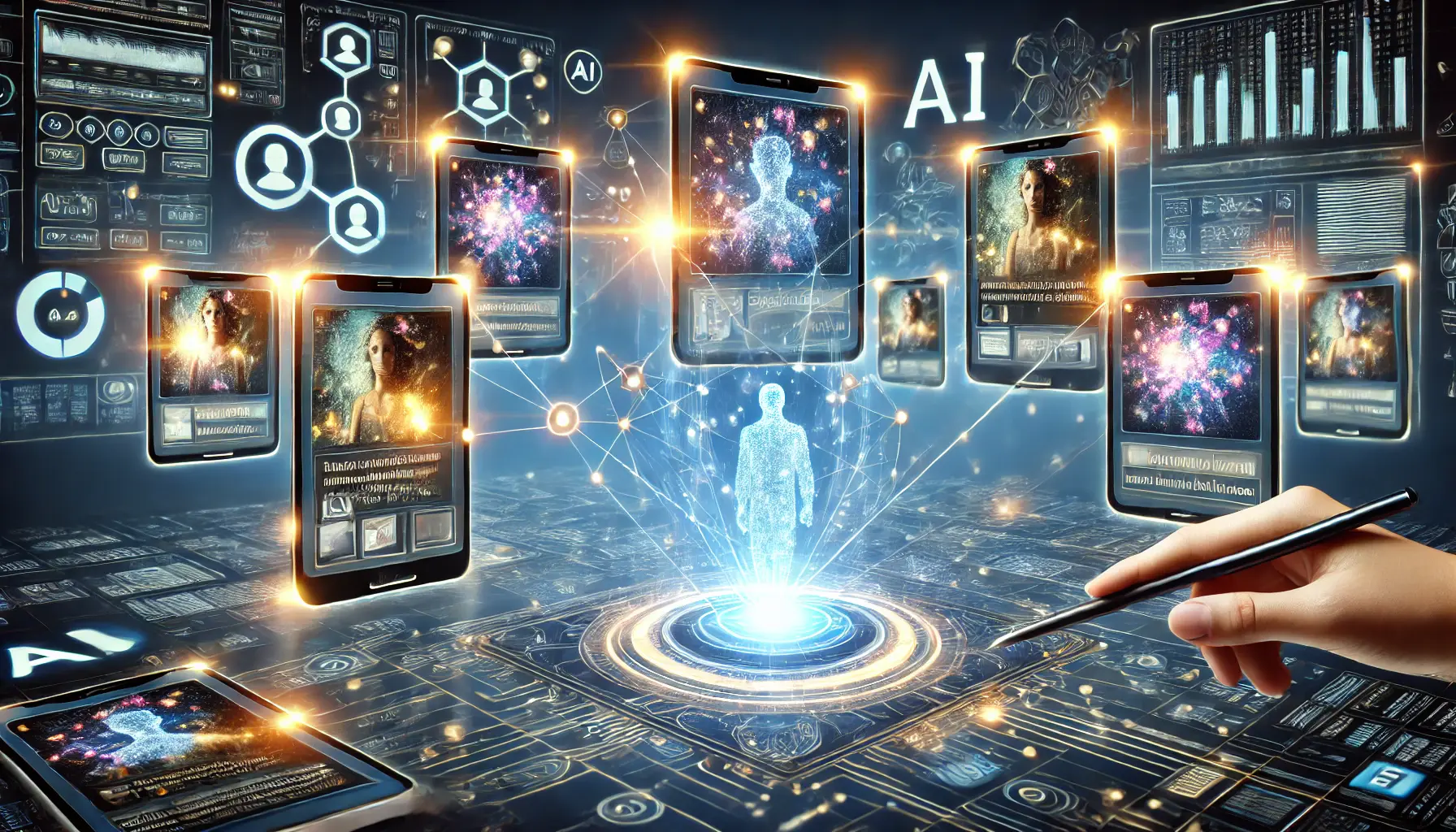
Visualizing the process of creating personalized ad content using artificial intelligence to enhance user experience.
Crafting Personalized Ad Content Through AI
Machine learning algorithms analyze user data to generate personalized ad content.
By understanding user preferences, behaviors, and demographics, AI can craft ads that align closely with individual interests.
- Content Customization: Adjusting ad visuals and messages to match user preferences.
- Product Recommendations: Suggesting products or services based on user browsing and purchase history.
- Localized Messaging: Tailoring ad content to reflect local cultures and languages.
Implementing AI-driven personalization ensures that users receive relevant and engaging ad content, leading to higher satisfaction and conversion rates.
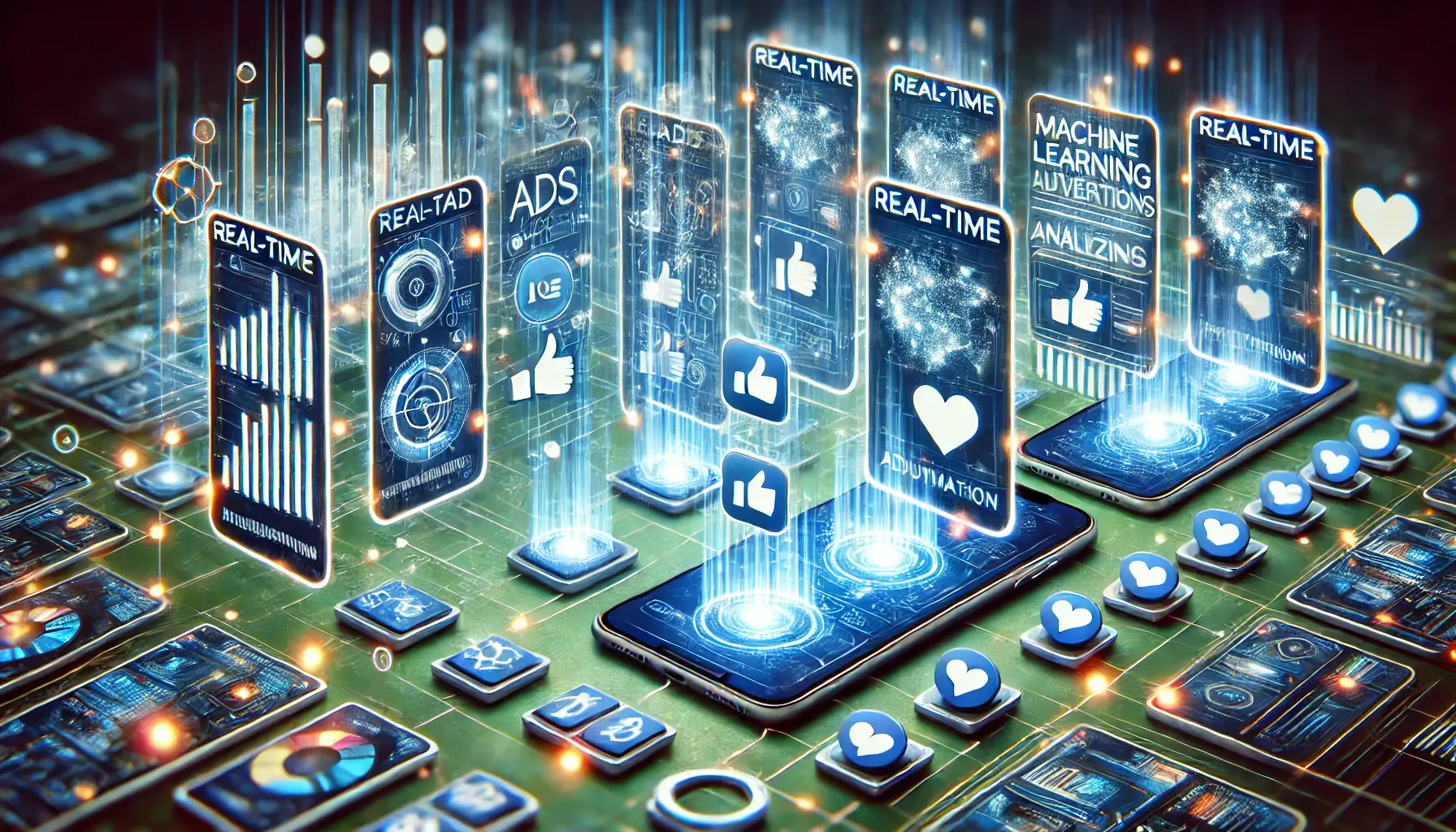
Visualizing how ads adapt in real-time based on user interactions, using data and machine learning algorithms for dynamic content adjustments.
Real-Time Adaptation of Ads Based on User Interactions
Machine learning enables ads to adapt in real-time based on user interactions.
AI uses the information derived from monitoring how users engage with ads to modify content to better suit user preferences.
- Behavioral Tracking: Monitoring user interactions to inform ad adjustments.
- Dynamic Content Updates: Modifying ad elements in real-time to enhance relevance.
- Feedback Integration: Incorporating user feedback for continuous improvement.
Real-time adaptation ensures that ads remain relevant and engaging, increasing their likelihood of being noticed and acted upon by users.
Dynamic ad personalization uses AI to tailor ad content, ensuring relevance and improving engagement rates.
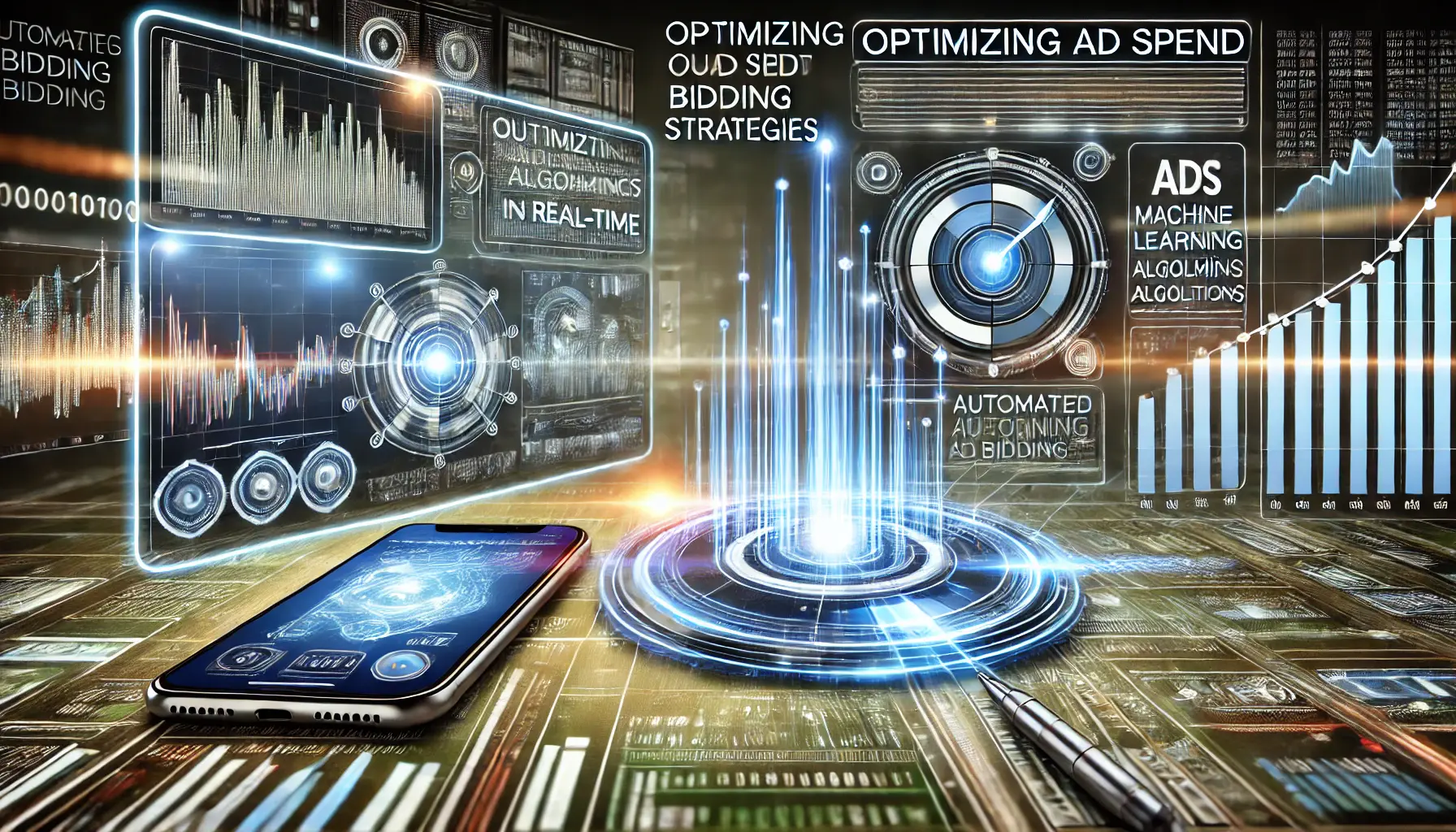
Visualizing the process of optimizing ad spend through automated bidding strategies with real-time adjustments and data-driven insights.
Technique 3: Optimizing Ad Spend with Automated Bidding Strategies
In the realm of app advertising, efficiently managing your ad spend is crucial.
Automated bidding strategies, powered by machine learning, offer a sophisticated approach to optimize your budget, ensuring your ads reach the right audience at the right time.
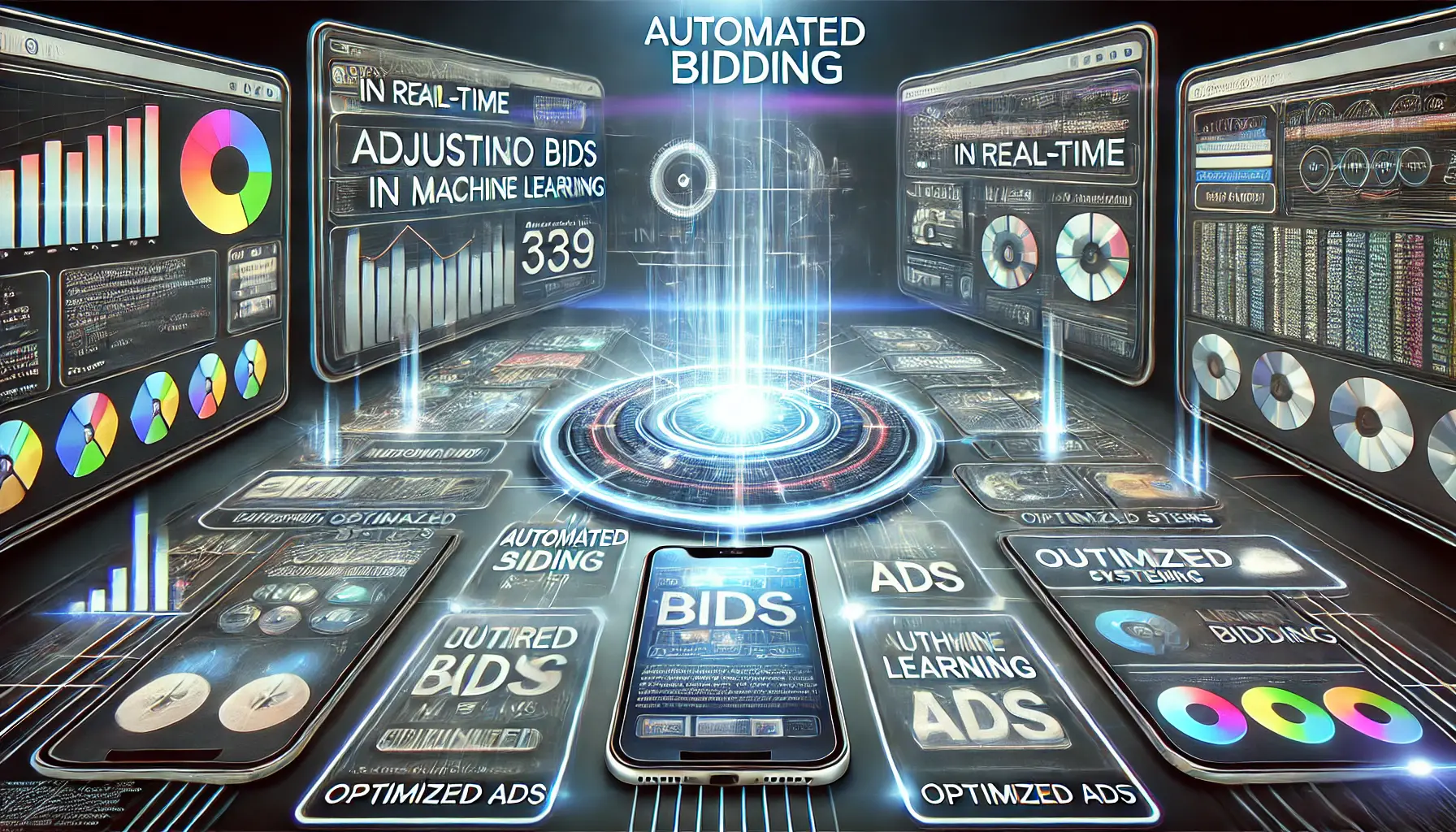
Visualizing the real-time adjustments of automated bidding in machine learning to optimize ad targeting and performance.
Understanding Automated Bidding in Machine Learning
Automated bidding leverages machine learning algorithms to adjust your bids in real-time based on various factors such as user behavior, device, location, and time of day.
This dynamic approach allows for more precise targeting and efficient use of your advertising budget.
- Real-Time Adjustments: Bids are modified instantly to align with current market conditions and user intent.
- Data-Driven Decisions: Machine learning analyzes vast amounts of data to inform bidding strategies, reducing guesswork.
- Scalability: Automated systems can manage large campaigns across multiple platforms without manual intervention.
By implementing automated bidding, advertisers can achieve better performance outcomes with less manual effort.
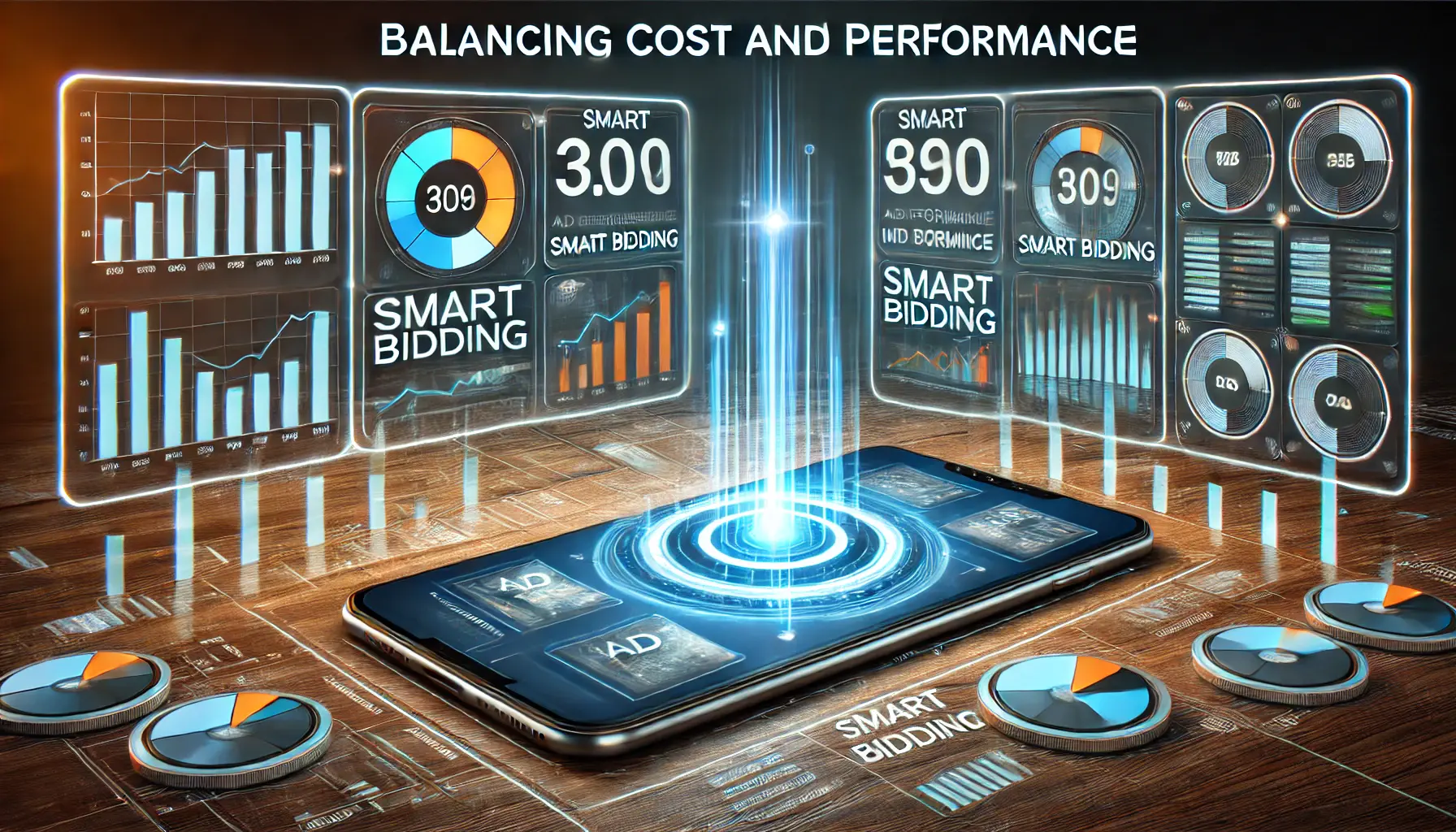
Visualizing the balance between cost-efficiency and performance in smart bidding through real-time optimization and data analysis.
Balancing Cost and Performance with Smart Bidding
Smart Bidding is a subset of automated bidding strategies that focus on maximizing specific performance metrics, such as conversions or return on ad spend (ROASReturn on Ad Spend, a metric to measure the revenue generated for every dollar spent on ads.).
Smart Bidding automatically adjusts bids by applying machine learning algorithms to optimize for your defined goals while keeping costs efficient.
- Target CPA (Cost Per Acquisition): Sets bids to help get as many conversions as possible for the target cost per action you set.
- Target ROAS (Return on Ad Spend): Adjusts bids to maximize conversion value and reach your desired return on ad spend.
- Maximize Conversions: Automatically sets bids to help get the most conversions for your campaign while spending your budget.
By leveraging Smart BiddingA subset of automated bidding that uses machine learning to optimize for performance metrics. strategies, advertisers can concentrate on strategic planning while all bids are automatically optimized by the system in light of performance objectives.
- Automated bidding strategies utilize machine learning for real-time bid adjustments.
- They ensure cost-efficient targeting and maximize ROI.
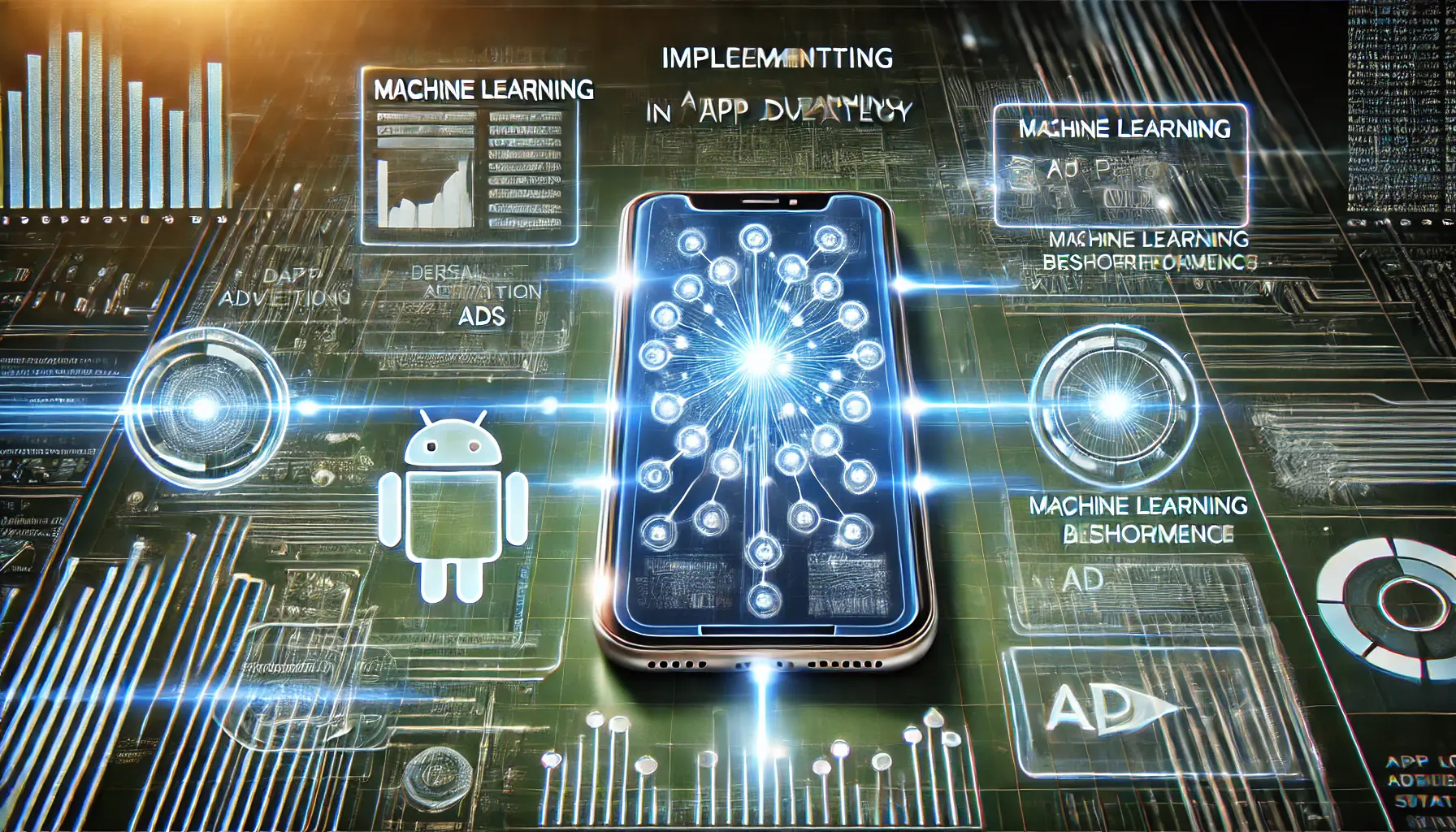
Visualizing the integration of machine learning into app advertising strategies, optimizing ad targeting and performance.
Implementing Machine Learning in Your App Advertising Strategy
Integrating machine learning into your app advertising strategy can significantly enhance campaign effectiveness.
By leveraging data-driven insights, you can create more personalized and efficient advertising experiences.
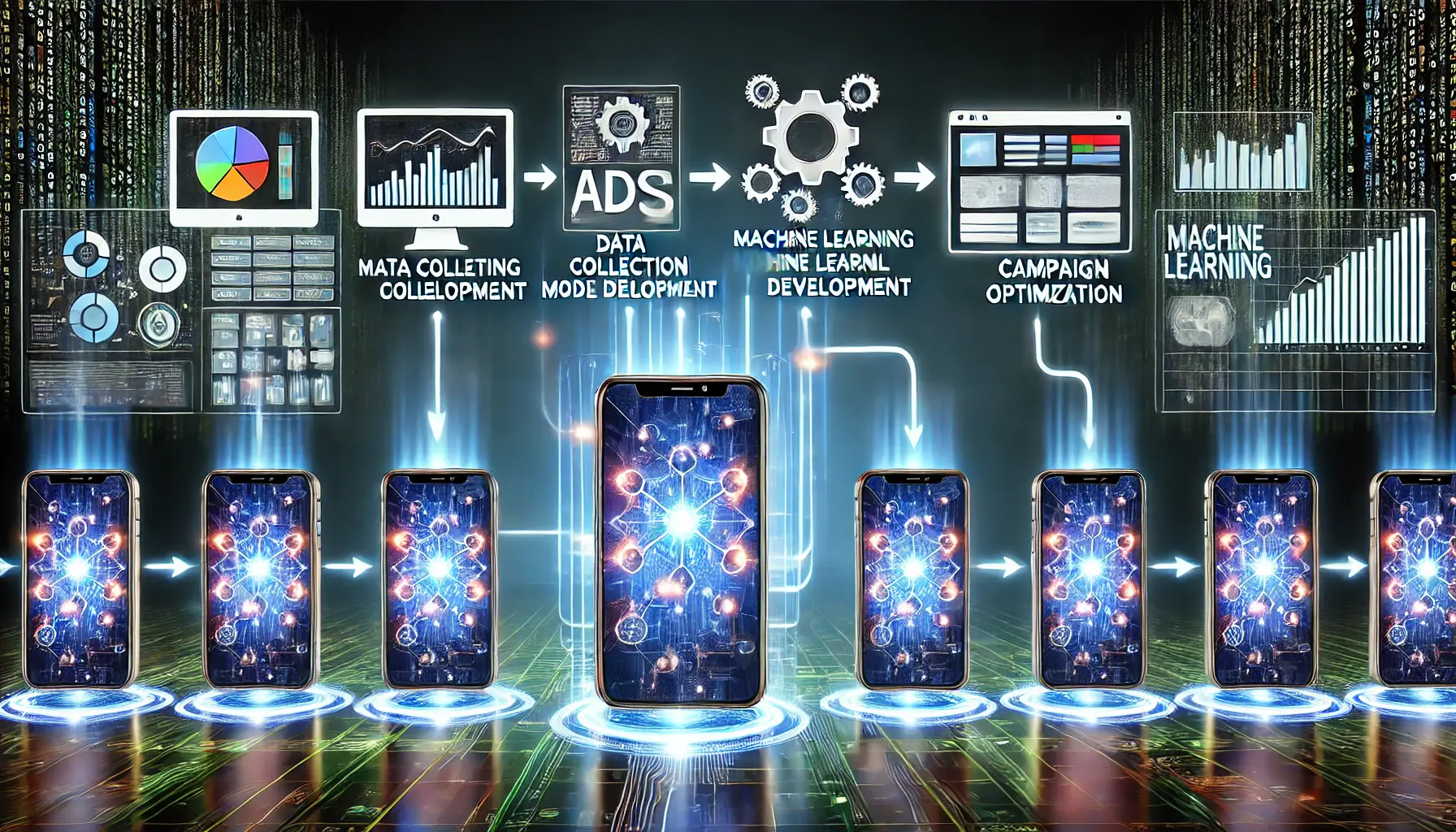
Visualizing the process of integrating machine learning into advertising, from data collection to campaign optimization.
Steps to Integrate Machine Learning into Your Advertising Efforts
To effectively incorporate machine learning into your advertising strategy, consider the following steps:
- Data Collection: Gather comprehensive user data, including demographics, behaviors, and preferences.
- Choose the Right Tools: Select machine learning platforms and tools that align with your advertising goals.
- Model Development: Develop predictive models to analyze user data and forecast behaviors.
- Campaign Optimization: Utilize insights from machine learning models to refine and optimize your advertising campaigns.
- Continuous Monitoring: Regularly monitor campaign performance and adjust strategies based on real-time data.
By following these steps, you can harness the power of machine learning to create more targeted and effective advertising campaigns.
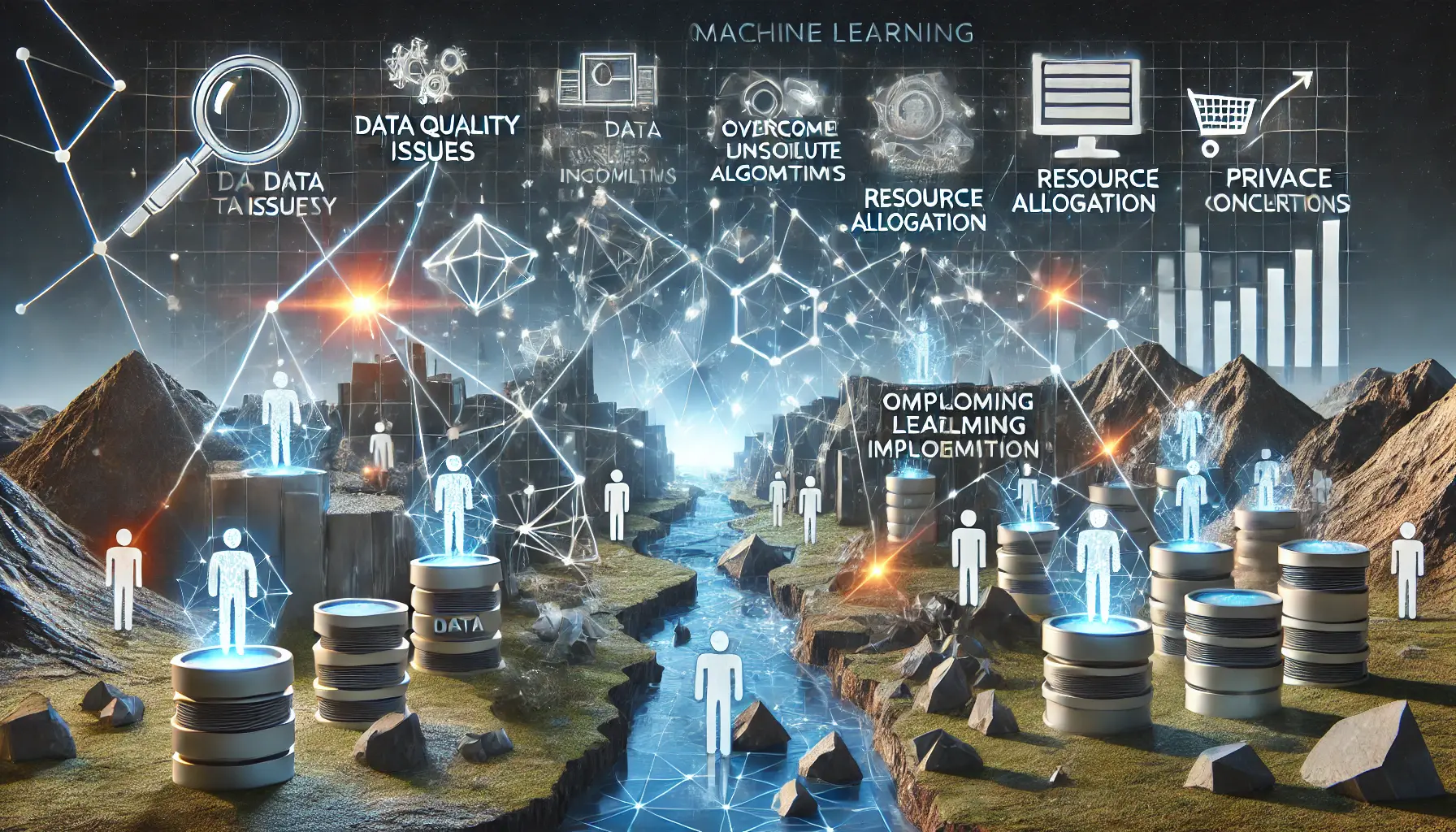
Visualizing the process of overcoming challenges in machine learning, such as data quality and resource allocation, in advertising strategies.
Overcoming Challenges in Machine Learning Implementation
While machine learning offers numerous benefits, implementing it in your advertising strategy may present certain challenges:
- Data Quality: Ensure the data collected is accurate, relevant, and up-to-date to train effective models.
- Resource Allocation: Implementing machine learning requires investment in technology and skilled personnel.
- Privacy Concerns: Adhere to data privacy regulations and ensure user data is handled responsibly.
- Model Complexity: Developing and maintaining complex models can be resource-intensive and may require specialized expertise.
By proactively addressing these challenges, you can successfully integrate machine learning into your app advertising strategy, leading to more effective and efficient campaigns.
Effective implementation of machine learning requires high-quality data, proper tools, and continuous monitoring to overcome challenges.
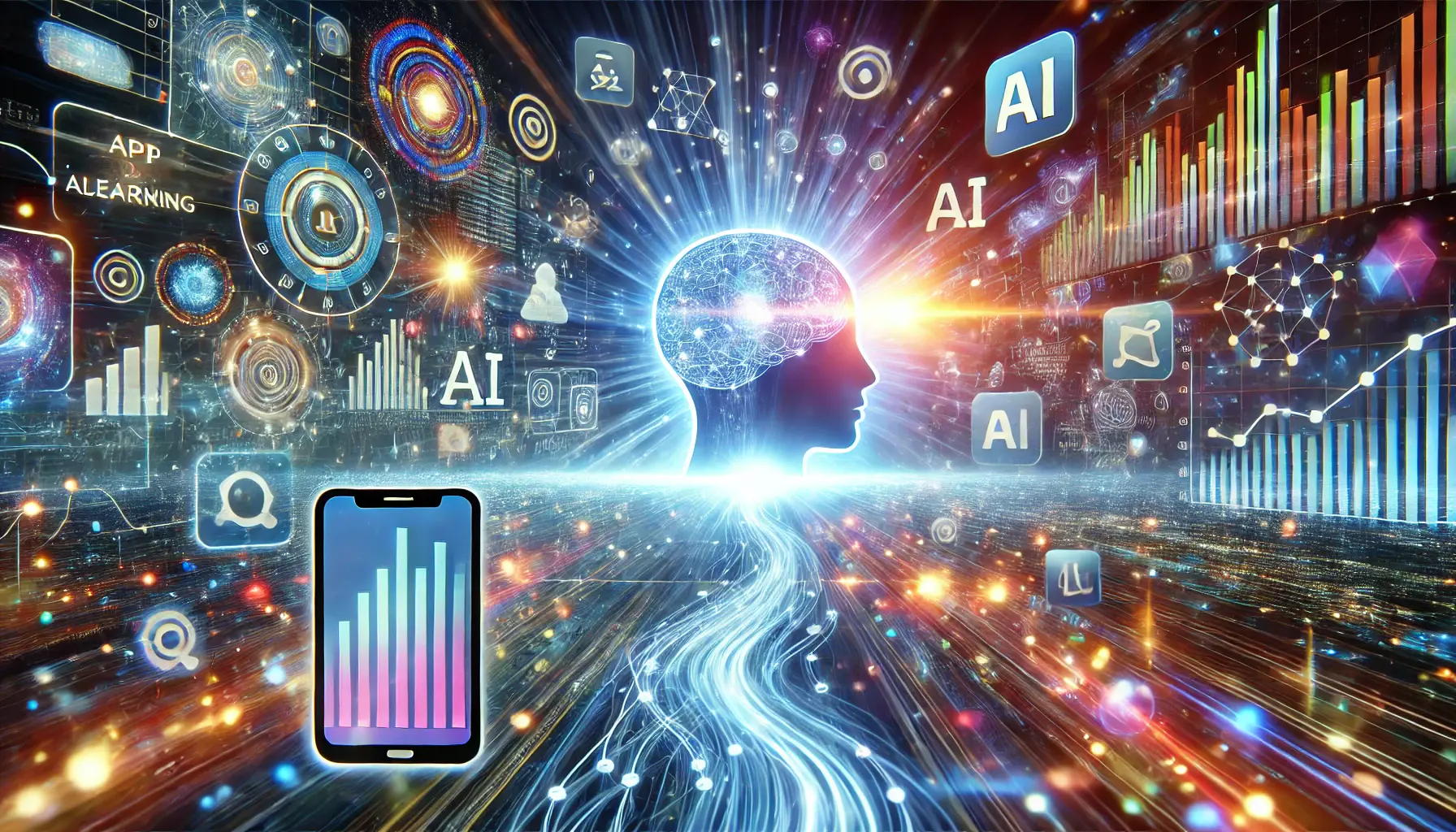
Visualizing the immense potential of machine learning in revolutionizing app advertising through data-driven optimization and personalized content.
Conclusion: Unlocking the Potential of Machine Learning in App Advertising
Machine learning has transformed the landscape of app advertising, empowering marketers to create more personalized, efficient, and impactful campaigns.
By leveraging data-driven insights, predictive analytics, and automation, app advertisers can connect with their target audience more effectively than ever before.
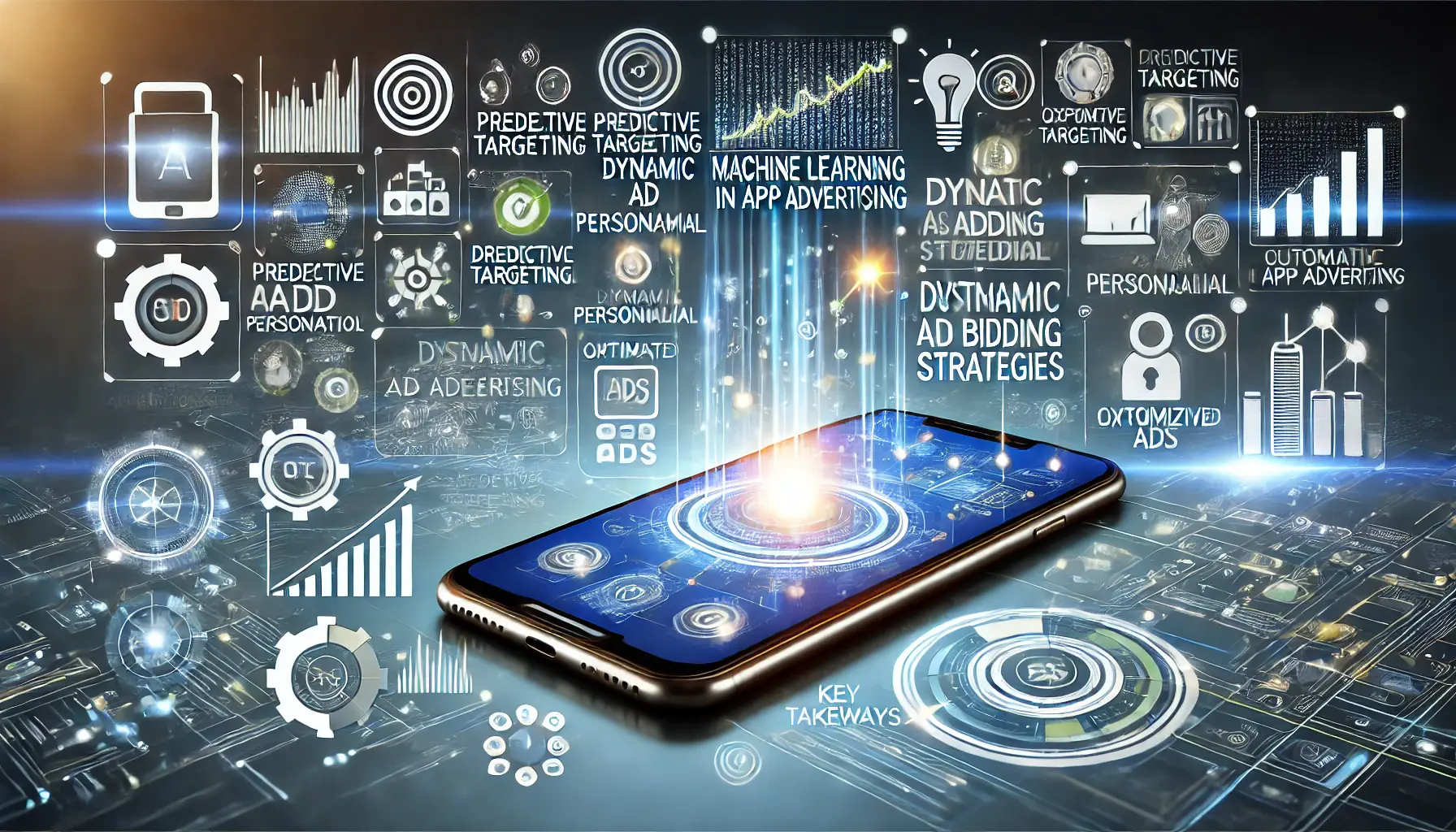
Visualizing the key concepts in machine learning for app advertising, including predictive targeting, dynamic personalization, and automated bidding.
Key Takeaways from the Article
Throughout this article, we explored several powerful techniques and strategies for integrating machine learning into app advertising.
Here are the highlights:
- Predictive Targeting: Machine learning algorithms enable advertisers to anticipate user behaviors and preferences, ensuring ads are tailored for maximum relevance and engagement.
- Dynamic Ad Personalization: AI-driven personalization creates unique ad experiences that resonate with individual users, fostering deeper connections and higher conversion rates.
- Automated Bidding Strategies: Smart bidding leverages machine learning to optimize ad spend, achieving better performance outcomes while saving time and resources.
- Strategic Implementation: From data collection to continuous monitoring, integrating machine learning requires a systematic approach to overcome challenges and maximize results.
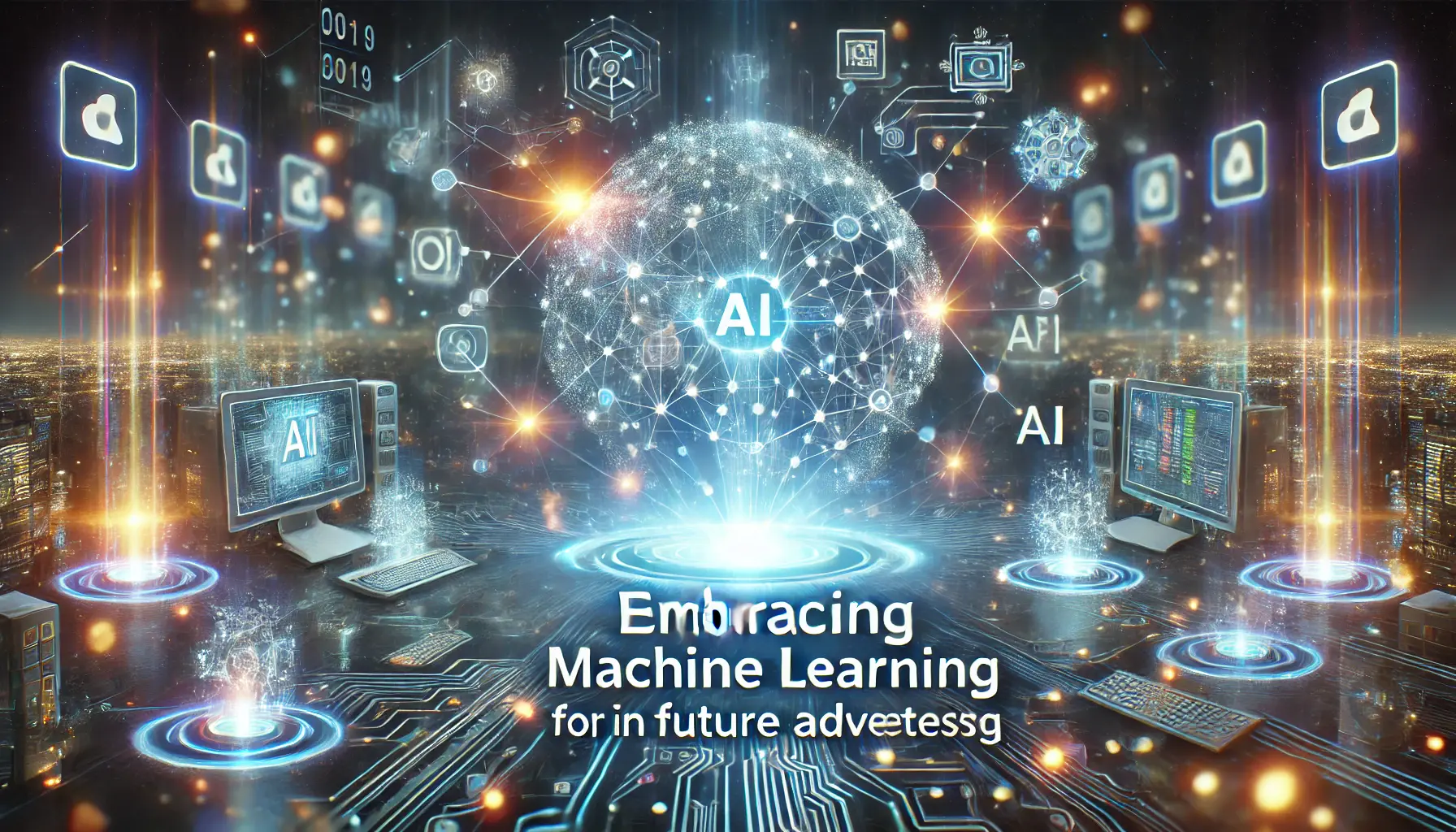
undefined
Embracing Machine Learning for Future Success
The future of app advertising lies in the hands of those who can harness the power of machine learning.
With increasing competition in the digital marketplace, these innovative strategies will be key to staying ahead.
By focusing on predictive analytics, dynamic personalization, and automated optimization, you can drive higher engagement, improve ROI, and deliver exceptional user experiences.
Start integrating machine learning into your app advertising strategy today and unleash its full potential.
With the right tools and a clear vision, your campaigns can achieve remarkable success in reaching your audience and achieving your business goals.
Machine learning empowers advertisers to create more personalized, efficient, and impactful campaigns, ensuring future success.
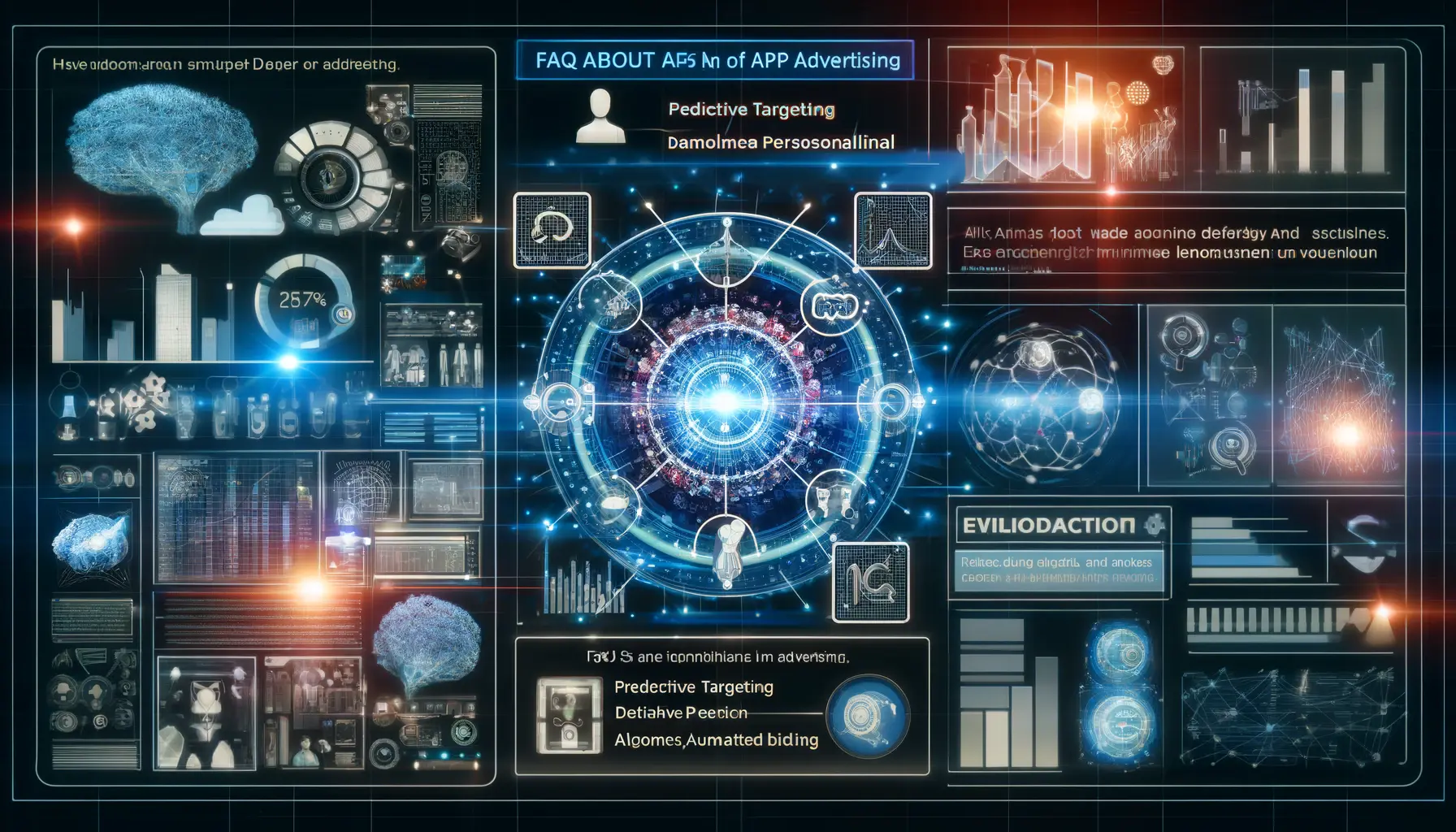
undefined
Your campaigns can be managed by an agency specialized in Google Ads, check out our service page.
FAQs about Machine Learning in App Advertising
As machine learning continues to revolutionize app advertising, several common questions arise.
Below are concise answers to some of the most frequently asked questions on this topic.
Machine learning for app advertising involves using algorithms to analyze user data, predict behaviors, and optimize ad campaigns to increase targeting and engagement.
Predictive targeting utilizes machine learning to anticipate user preferences, enabling advertisers to serve more relevant ads and enhance user engagement.
Dynamic ad personalization tailors ad content to individual users, leading to higher engagement rates, improved user experiences, and increased conversion rates.
Automated bidding strategies utilize machine learning to adjust bids in real-time, ensuring optimal ad placements while maximizing return on investment.
Challenges include ensuring data quality, effective resource management, addressing privacy concerns, and managing the complexity of developing and maintaining machine learning models.
Begin by gathering substantial user data, selecting appropriate machine learning tools, developing predictive models, optimizing campaigns based on insights, and continuously monitoring performance.
While machine learning offers significant benefits, its suitability depends on factors like available data, resources, and specific advertising goals.
Machine learning relies on user data, making it essential to adhere to privacy regulations and handle data responsibly to maintain user trust.
High-quality data is crucial, as machine learning models depend on accurate and relevant data to make effective predictions and optimizations.